In this blog post our partner SciBite explains how they integrated Linkurious with their solutions to open new possibilities in the research and treatment of rare diseases.
SciBite’s “Semantics as a Service” platform transforms unstructured text (documents, news, patents etc) into structured, insightful data. The system comprises:
- Highly curated scientific ontologies, built upon established open standards
- Formal based Named Entity Recognition
- Relationship mapping and extraction, identifying patterns between concepts such as drugs, adverse events, companies, institutions and much more
- A user-friendly interface built on Elastic Search
- Web services that provide live enrichment of text content
- Seamless connectivity to third party applications, transforming search and connectivity
SciBite provides pluggable technology, so that you can integrate semantic enrichment exactly where you need it.
Fast, lightweight and simple to use, we transform data by providing technologies that understand the scientific content they process.
We focus on customers in the life sciences (pharmaceuticals, biotechnology, agrochemicals, food and consumer goods), including many of the world’s top pharmaceutical companies.
Rare Disease Day took place on 28 February, and to help publicise it, we thought we’d raise awareness of the kind of work happening in this area within the pharmaceutical industry, and how big data tools are impacting those efforts.
Incentives are now in place to encourage pharmaceutical companies to develop treatments for rare diseases (so called orphan drugs), making this a real area of importance for the industry and patients.
It’s still a costly and long journey though, with a number of hurdles along the way. We wanted to show how SciBite’s technology can help pharma to overcome them. The three biggest issues for researchers in this area are:
- Finding other researchers working on relevant areas for collaboration
- Faster, deeper research into the mechanistic behaviour of rare diseases
- Linking rare diseases through shared phenotypes
Our aim is to develop technology which empowers scientists to address those hurdles above.
Graph technologies enable us to link complex data from multiple sources, in a flexible extensible manner.
Compared to a relational database approach, graph technologies promote a more fluid linking between data sets. This then renders the data more accessible through being much easier to read, at a glance. There’s also the advantage of more efficient joining across multiple data types.
SciBite has worked on linking drugs with adverse events and diseases with phenotypes. In all of these projects, we’ve had to pull together data from different public repositories, each with their owns standards and naming conventions. The big challenge is bridging the gaps between disparate data sets – when we bring SciBite’s technology together with graph visualisation, we’re able to query across all of these previously disconnected datasets.
We pulled data from a couple of sources:
- For collaboration networks, we analysed address fields from Medline – a bibliographic database of life sciences and biomedical information – and overlaid geographical location data
- For looking into the mechanistic behaviour of rare diseases, we automatically scanned the whole of Medline for disease phenotype co-occurrences and then we applied statistical analysis to compare diseases based on their phenotype profiles.
A simple representation of the graph model shows nodes representing diseases and phenotypes, with edges weighted by the strength of the relationship between pairs of nodes:
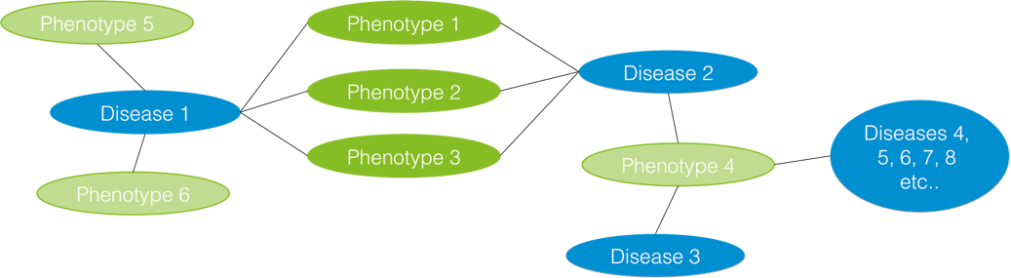
This was abstracted further to enable direct connections between diseases via a “relatedness” score, based on their shared phenotypes. From there it is possible to lead some of the following investigations.
For this example we focused on Friedreich’s Ataxia, a rare condition that causes progressive damage to the nervous system, caused by a deficiency in frataxin protein (FXN). As is the case with many rare diseases, little is known about how its underlying mechanisms, so we wanted to get an idea of where FXN might fit in with the other gene/protein entities displayed on the graph below, within the context of looking deeper into Friedreich’s Ataxia.
Through adding in protein-protein interaction data from iRefIndex, a consolidated protein interaction database, it was possible to fill in some of the gaps around the molecular interactions of FXN and how this links to conditions with visible similarities to Friedreich’s. From the graph, we can see FXN interacting with several genes that are known to be associated conditions that show a similar set of symptoms. Through building up a picture of related conditions and their common molecular mechanisms, we provide a tool to support experts in the field with their research to gain a deeper understanding of the disease.
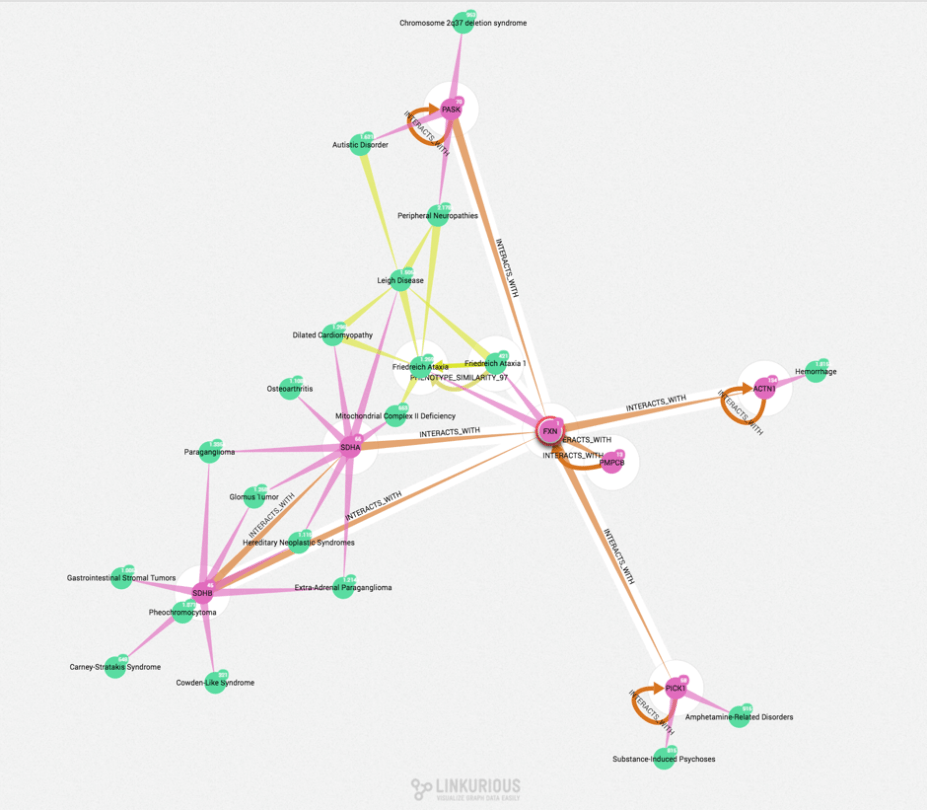
The incredibly useful thing about this method is that we’ve brought together three sets of data:
- Diseases related by a similar set of clinical signs automatically identified from Medline documents (courtesy of our text analysis engine, TERMite) – seen here in yellow lines
- Gene disease associations from DIsGenet (a database of gene-disease associations) – pink lines
- Protein-protein interaction data from Irefindex – orange lines
For this project, we pulled in drug side effect reports from the FDA Adverse Event Reporting System (FAERS) and linked these up with their major molecular targets (i.e. the things to which drugs bind in order to show a pharmacological effect). It was a great way to cut through a very complex dataset and get an overview of drugs that share common side effects and how these side effects might be associated through particular molecular targets. So, with this graph structure in place, we can ask questions like “What are the major side effects of drugs which are AGTR1 antagonists?” This wouldn’t have been possible without an effective way of knitting together two disparate data sources.
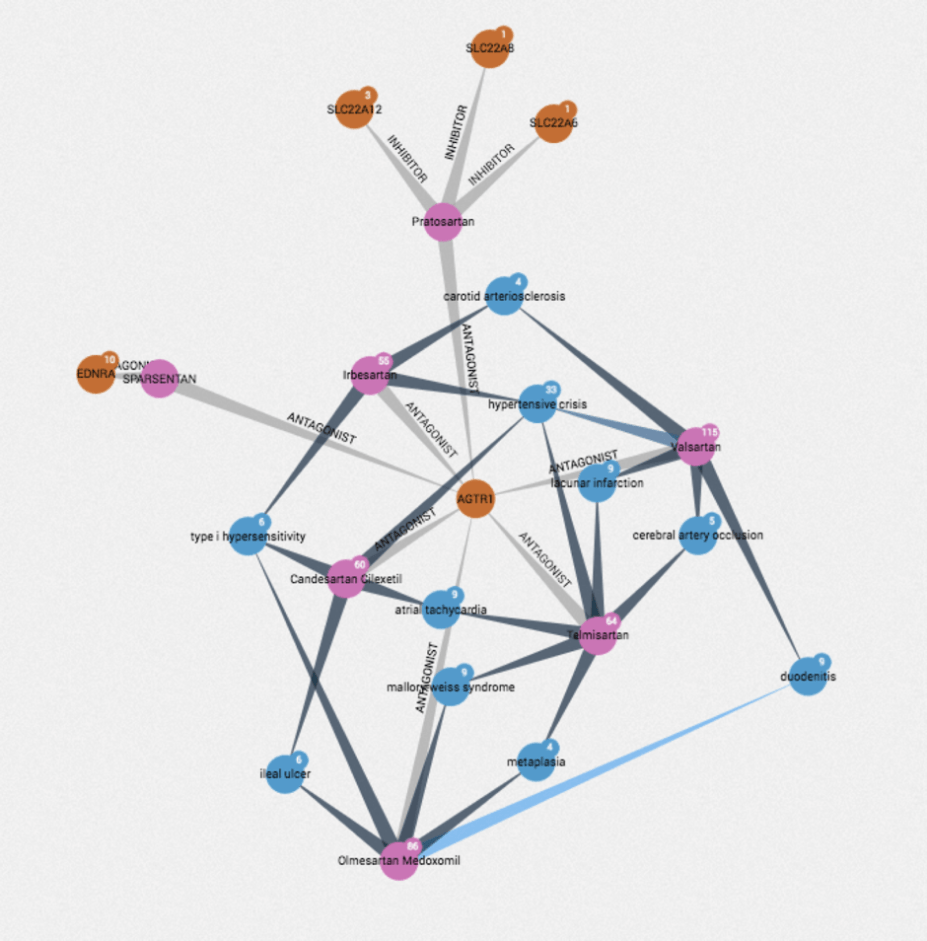
For example, the image above shows AGTR1 molecular target (orange) linked to “duodenitis” side effect (blue) via two separate antagonist drugs, in this case, Olmesartan medoxomil and Valsartan (pink). This starts to build up evidence of a wider relationship between drugs that are AGTR1 antagonists and the possibility of duodenitis.
As you can imagine, scanning 26 million Medline abstracts results in a fair amount of data (to say the least!). To be precise, >45k unique nodes representing diseases, genes and phenotypes with >2.5 million connections between them. In order to answer specific scientific questions, the challenge is to cut through the noise and focus on only the more interesting parts of the graph.
Essential to this process was the way Linkurious enabled us to start from a single node and then interactively build out a graph from there. With the sheer number of nodes and relationships, the potential for a “hairball” effect was high and the Linkurious interface helped avoid this. Additionally, the embedding of the Cypher query language in the platform enabled us to run more complex queries that were required to generate a useful visualisation.
We’re always looking at new ways of linking data. This could be helping to further pharmacovigilance work, exploring food for its therapeutic benefits, or looking at adverse events associated with drugs or cosmetics.
Contact SciBite on info@scibite.com for more information or to discuss your project.
You can also try Linkurious demo for free!
By Michael Hughes, Senior Informatics Scientist at SciBite.
A Biologist at heart, Michael has spent his career mining for gold in scientific literature. At SciBite since 2015, he’s now part of a global movement to transform data and how we interact with it.
A spotlight on graph technology directly in your inbox.