Graph Viz 101: Emergence of knowledge through visualization
Graph Viz 101 is a series of posts to teach the basics of graph visualization, written by Sébastien Heymann in collaboration with Bénédicte Le Grand of Université de Paris 1. This is our third post, please discuss it below!
In this blog post we provide a short introduction to the emergence of knowledge through visualization.
The goal of Exploratory Data Analysis is to find the best hypothesis which supports the observation of data. The knowledge discovery process is thus considered to be abductive, i.e. given an observation, our explanation has a reasonably good chance to be right according to our current results, knowledge, and intuition, but there might be an unknown number of explanations that can be at least as good as this one. Further studies through visualization and statistical analysis are then necessary to try disproving our explanation in favor of a better one. The explanation may finally be accepted after a couple of experiments that fail at invalidating it. The insights gained may be used to confirm already known results, as well as provide ideas of novel statistical indicators and data descriptors.
The data properties spotted by visual saliences may challenge current hypotheses and raise new questions. The analyst may want to modify the visualization accordingly, to eventually select a picture which clearly reveals an issue, or which supports a hypothesis. The key role of visualization in the emergence of knowledge is emphasized in (Tukey 1977):
« The greatest value of a picture is when it forces us to notice what we never expected to see. »
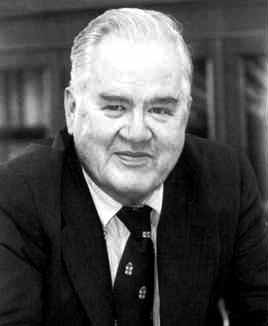
We illustrate it on a simple example: in the distribution of file sizes in a P2P system (see figure below), we observe clear peaks on specific values, and we know that these values correspond to the most common sizes of films, depending on their formats. There values are thus interesting outliers, not
anomalies in data.
We may then raise the following hypothesis: Even though in principle files exchanged in P2P systems may have any size, their ctual sizes are strongly related to the space capacity of classical exchange and storage supports.
The visual investigation of this P2P dataset helped the authors of the study to make a discovery, which however had to be confirmed by complementary analyses.
In the next post we will talk about the visual representation of graphs.
Sébastien
A spotlight on graph technology directly in your inbox.