Fighting financial crime is a daily battle worldwide for banks and other regulated businesses. Organizations deploy intelligent systems to prevent and detect suspicious activity, such as anti-money laundering (AML) control frameworks. We’ll see in this blog post how graph technology can reinforce AML analysis systems.
In today’s complex economy, law enforcement and financial organizations fight against a wide range of financial crimes: embezzlement, tax evasion, extortion, corruption, sanctions evasion, fraud, or money laundering, to name a few. While tracking down those activities, governments and financial institutions must deal with a fast-moving financial crime landscape and a growing volume of information of various formats.
Graph technology can be a powerful asset to help fight financial crime. It provides exhaustive overviews of the different entities and their connections. And it supports complex pattern queries on large data-sets in a near-real-time environment.
In this article, we’ll focus on anti-money laundering procedures and explore a specific case with a graph analytics approach.
Governments have been steadily strengthening AML rules to prevent money laundering activities. Financial institutions are now required to follow strict AML programs, to practice constant monitoring for suspicious activities, and to report on any suspected cases of money laundering. There is increased regulatory pressure, and compliance failures might be penalized with large penalties.
Analysts within financial institutions rely on risk-based AML analysis frameworks to monitor their customers and their financial transactions. But professionalized criminals deploy sophisticated tactics to hide their wrongdoings. They use shell corporations, tax havens, and complex financial fraud schemes to prevent identification or tracking of money flows. To thwart such criminal strategies, finding information about a specific suspicious entity is not enough. Financial crime units must investigate the connections between individuals, accounts, companies, locations, to trace complex transactions. This is why network analysis and visualization technologies have proven to be efficient tools to support AML analysis processes.
We explore below how to use a graph-based investigation platform such as Linkurious Enterprise to monitor high-risk customers through various layers of data.
Banks and other financial institutions keep track of numerous sources of information about their customers (both individuals and businesses) and their financial activities. Graph technology enables organizations to index complex connected data and easily query them to find patterns. With such systems, organizations can bring together various information into a single data model.
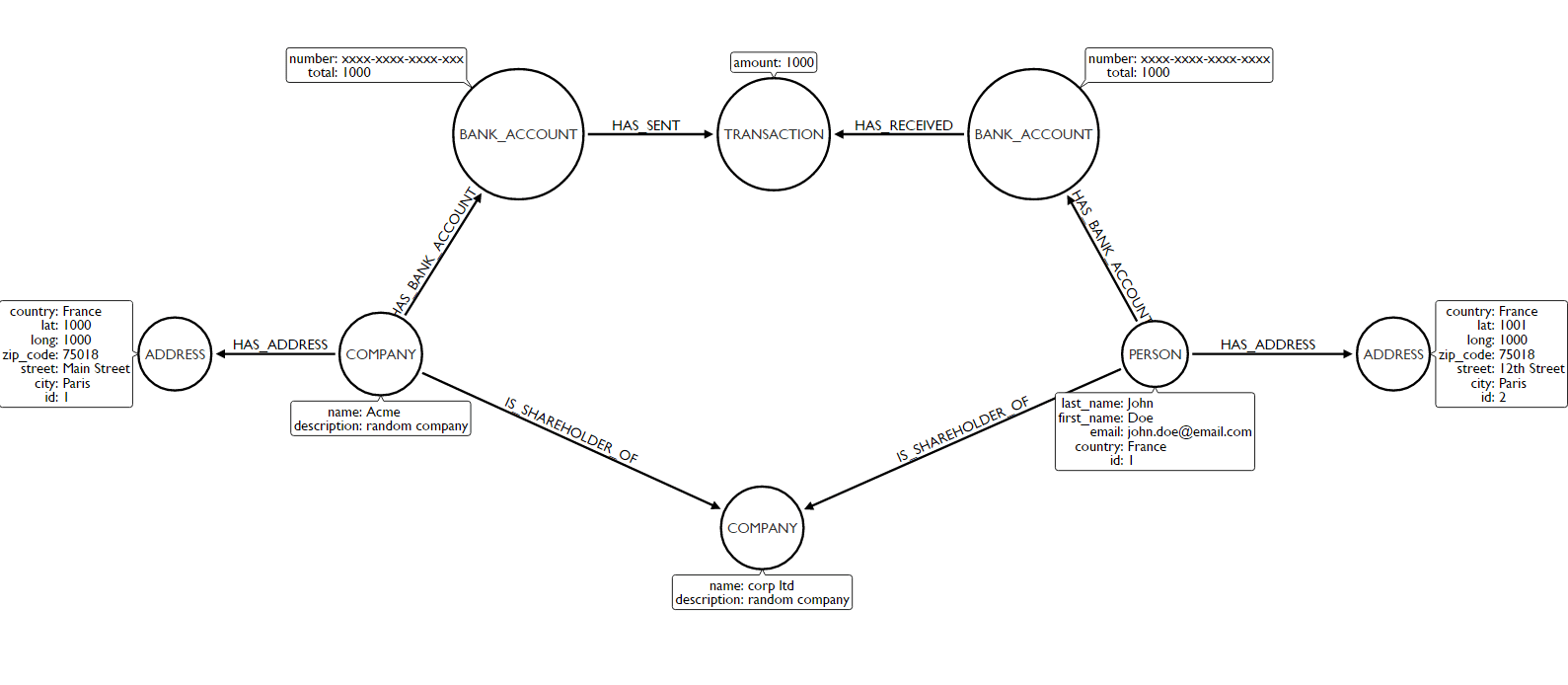
Linkurious Enterprise provides an advanced graph visualization and analysis software compatible with numerous graph databases to easily explore and monitor the data.
AML regulations require banks to monitor their high-risk customers. Listed on special watch lists, those individuals can be identified either by authorities (e.g via a politically exposed person list or Specially Designated National Lists) or by the institution itself (e.g customers with repeated suspicious transactions).
“Are my customers currently involved in activities with flagged individuals?” “If yes, are these activities suspicious?” Organizations need to be able to answer those questions.
Linkurious Enterprise provides analysts with an interface to monitor high-risk patterns in real time. They can set up alerts for specific patterns with a graph query language like Cypher. For instance, an AML analysis specialist might want to be warned each time there is any type of connection between my customer’s financial activities and their watch list. She can use the following query to create an alert in the system:
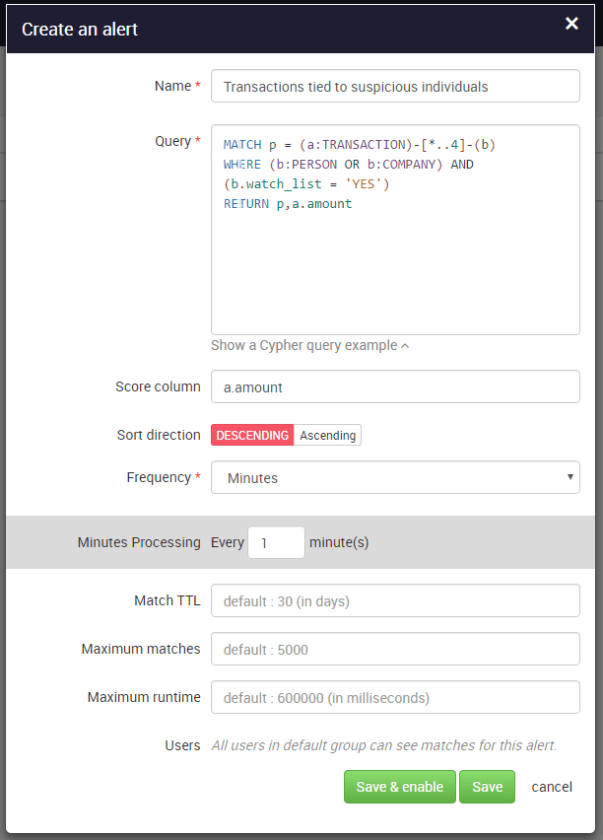
If new data is added to the database (e.g. transactions, persons, companies or relationships), Linkurious Enterprise will automatically search for and flag suspicious connections. With the graph visualization interface, it’s then easy to investigate and assess the different cases.
The alert system reported several matches to our query. To evaluate the risk-level of the cases, analysts can take advantage of the visualization interface to quickly visualize and investigate the alert (we talk in another article of the benefits of graph visualization). Let’s take a look at one of the alert match:
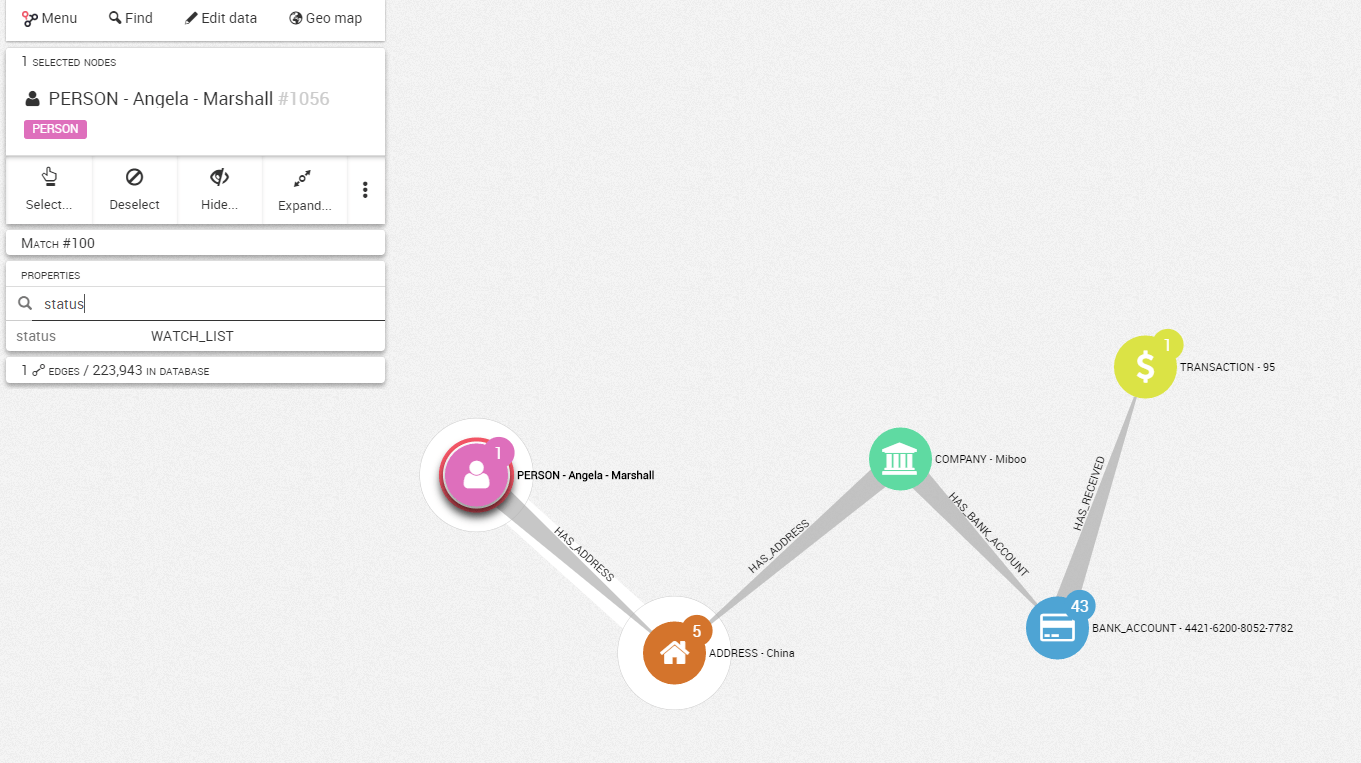
At a glance, you can see that Angela Marshall (a fictitious individual who belongs to my watch list) is indirectly connected to a transaction on a customer’s bank account. She appears to share the same address as a customer, the company Miboo.
This pattern is relatively suspicious. The analyst might want to explore beyond this single connection and see which other entities are linked to this address.
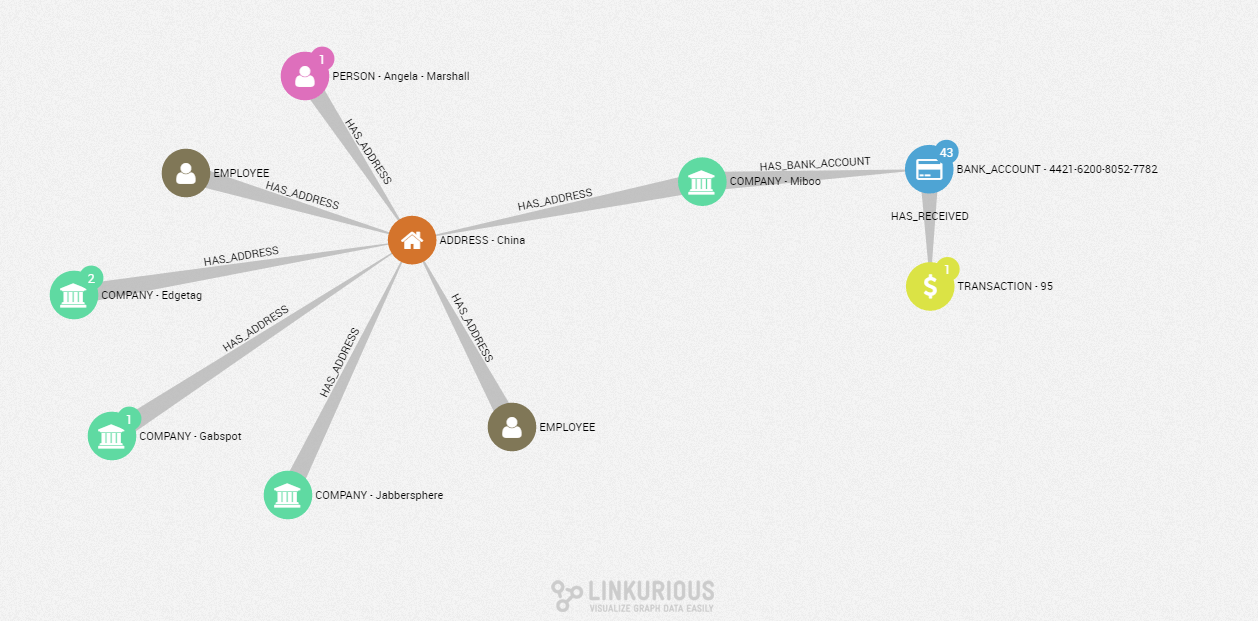
In addition to sharing an address with an individual on a watch list, this suspicious customer also shares his address with three other companies and two of our internal employees. They are all living in the city of Hongqiao, China on 3557 Straubel Circle.
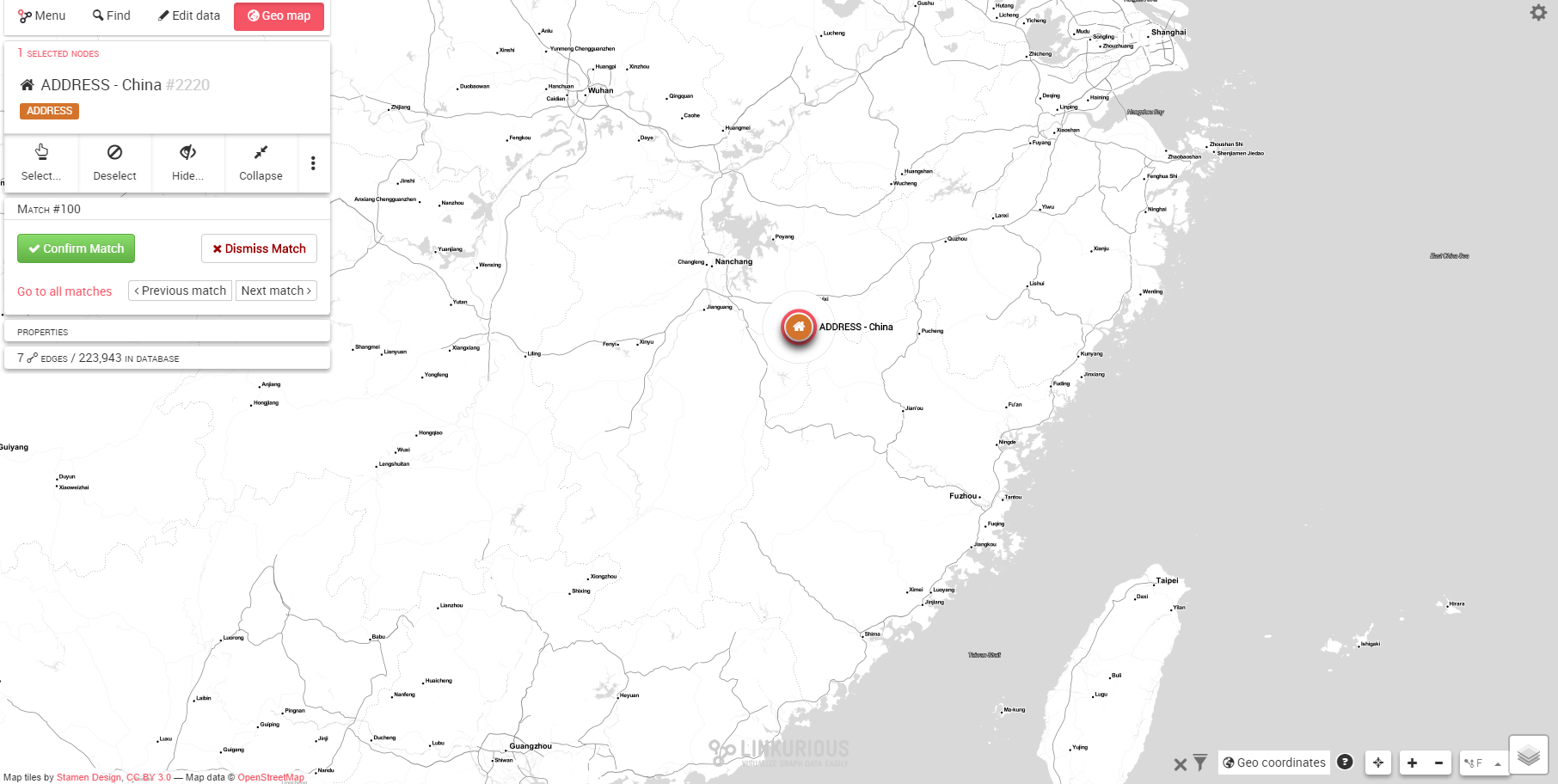
The analyst might recognize a known pattern of money laundering: different companies registered with a unique address. Also, some employees are connected to a known high-risk customer. This information can be reported to higher authorities for further investigation.
Graph analysis focuses on relationships and therefore helps to discover hidden connections between different entities. Linkurious Enterprise also operates an alert system in a near-real-time environment. That way, financial crime units can identify suspicious activity schemes instantly and reinforce their AML analysis and regulation system.
Learn more about how graph analytics can act as a powerful asset for more efficient financial crime detection and investigation in our white paper.
A spotlight on graph technology directly in your inbox.