With the combined forces of increased digital transformation and the global pandemic, consumer habits have shifted rapidly over the past few years. In the retail industry, buy now, pay later (BNPL) short term online lending has gained enormous momentum. Platforms like Klarna, Affirm, Zip, Flex, and Afterpay are offering a new way for consumers to pay for purchases ranging from clothing to furniture to groceries and more. Even Apple announced it is launching its own BNPL service.
BNPL has seen over 300% year on year growth in the US between 2018 and 2021 (1). And the BNPL sector is expected to top $1 trillion in annual gross merchandise volume by 2025 (2).
But BNPL also attracts fraudsters, who are experts at exploiting new products that may not benefit from the same robust security as more established payment methods.
This article explores what exactly makes short term lenders vulnerable to fraud, as well as the types of fraud BNPL providers typically face. We’ll also look at how network analytics are a key piece of the puzzle for more efficient fraud detection and investigation.
Buy now, pay later, or BNPL, lets customers pay for goods in increments. Instead of paying for a purchase in full upfront, payment is broken down into smaller chunks, without interest. These are repaid in installments. The BNPL provider pays the merchant in full upfront and then takes care of collecting those installments.
BNPL has been so successful in large part because it lets customers obtain goods without having to pay the entire cost at once. It also provides an alternative to using credit cards.
Buy now, pay later fraud has become a rising problem as this new means of payment has increased in popularity.
Besides the fact of being a new type of payment, several elements make BNPL especially vulnerable to certain types of fraud.
First, credit decisions are made in real time using internal algorithms that work on available customer information. This speedy approval is a must for BNPL platforms to deliver the easy user experience customers expect. But this also makes it easier for bad actors to slip through the cracks.
Second, the way BNPL payments are structured creates more opportunities for attack. A traditional e-commerce transaction is done through a single payment. BNPL payments on the other hand may take place across 4, 8, or 10 transactions.
Finally, buy now, pay later platforms conduct few formal credit checks. Banks and traditional creditors typically run in-depth checks on customers before granting them credit. BNPL providers instead rely on algorithms and scoring engines to perform credit checks. If the algorithms aren’t perfectly tuned to the tactics fraudsters are using, it can open the door to increased fraud risk.
Buy now, pay later has proven to be especially vulnerable to certain types of fraud: account takeover fraud, synthetic identity fraud, and new account fraud. None of these fraud schemes are unique to BNPL, but the nature of this type of payment makes it susceptible to these attacks.
A synthetic identity is a false identity mixing real and fake personal information. It may include a real address and a fake name, for example. This information together does not belong to any one real person. Using a synthetic identity makes it easier for a fraudster to get away with wrongdoing.
A fraudster using a synthetic identity when purchasing goods with BNPL will receive those items immediately, but has no intention of repaying. Using a synthetic identity, the fraudster is nearly impossible to track down since their personal information doesn’t match with any single real person.
Account takeover fraud, or ATO, is when an unauthorized user gains access to a victim’s account using the account’s login credentials. The account details may be purchased on the dark web or obtained through phishing or other forms of social engineering. The fraudsters then steal assets or personal information, or may even sell access to the account itself.
With BNPL, the fraudster uses the stolen account information to buy items that he will receive in the place of the account holder. The victim may only realize they’ve been scammed once the payments start to come due on their account weeks or even months later.
New account fraud is when a fraudster successfully opens a new account at a bank or payment service for the purpose of committing fraud. Everyone has a new banking or payments account at some point - even multiple new accounts - and most of these are legitimate. But some people open an account with the intention of wrongdoing.
With BNPL, it’s easy to open an account or access payment services. This helps create a seamless customer experience. But it also means that some fraudsters will try to open new accounts with the intention of using them to steal goods.
Network analytics, or graph analytics, is a powerful component of any tech stack used to detect and investigate BNPL fraud. Graph technology analyzes not only the individual data points but also the relationships between them. It’s perfectly suited to understanding and analyzing large networks of people, places, transactions, etc. Graph is highly scalable, letting you quickly analyze even very large datasets.
When applied to buy now, pay later fraud, network analytics lets you see the full network around clients rather than considering them in isolation, enabling you to analyze suspicious connections between multiple data points and investigate possible fraudulent activity more efficiently.
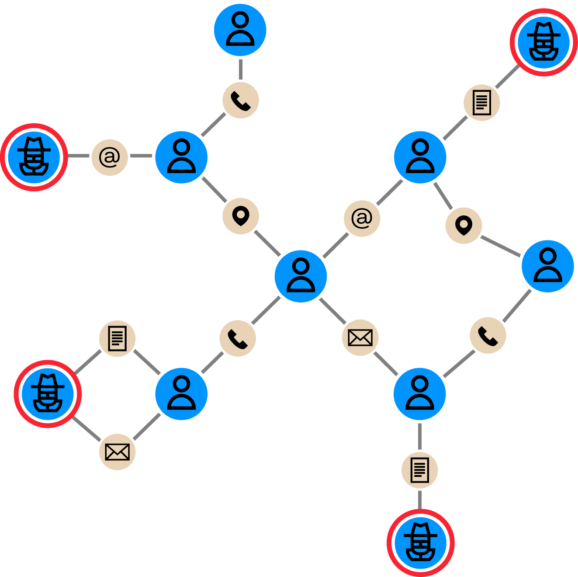
Say that as a BNPL provider, your company is facing networks of fraudsters acquiring loans through synthetic identities and then not paying them back. You have a risk scoring engine that can score and reject applications, but you’re not sure of the efficiency of the rules. Using a graph analytics tool like Linkurious Enterprise, you can look for communities of credit requests through personally identifiable information (PII) and digital footprints.
By visualizing rejected credit requests and their direct and indirect connections, you can quickly identify fraudsters that may have duped the risk scoring engine. And that information can then be fed back into the scoring engine to strengthen anti-fraud defenses.
Want to go deeper? Watch a demo about how to use graph analytics to expose BNPL fraud rings:
A spotlight on graph technology directly in your inbox.