Even the best preventive measures can’t stop all fraud. Banks and other organizations need to be ready to expect the unexpected as fraud techniques evolve as new opportunities arise. An effective fraud detection system is an essential piece of any anti-fraud strategy. Efficient fraud detection helps prevent losses, creates better customer experiences, and helps an organization build a better, more secure business.
In this article, we’ll take a look at what fraud detection is, and why it can be so challenging in our current context. Then, we’ll take a look at how financial institutions, governmental agencies, and other organizations work to detect fraud, from the more traditional detection techniques to newer, more cutting-edge technology solutions.
Fraud detection is the process of identifying and preventing deceptive activities across various domains, particularly financial transactions. It employs a combination of analytical methodologies, monitoring systems, and technological tools to spot patterns, irregularities, and anomalies that might indicate fraudulent behavior.
Modern fraud detection systems typically rely on advanced technologies like machine learning, artificial intelligence, and advanced analytics to analyze vast amounts of data in real time, flagging suspicious activities based on predefined risk indicators. The ultimate objective of fraud detection is to safeguard organizations and individuals from financial losses, protect sensitive information, maintain operational integrity, and ensure compliance with regulatory requirements while preserving trust in systems and institutions.
Fraud is an increasingly acute problem with fraud losses in many places growing year on year. In the United States, for example, the Federal Trade Commission (FTC) estimates that consumers lost $12.5 billion to fraud in 2024, up 25% compared to the previous year. And organizations globally lose up to 5% of revenue to fraud each year, according to the Association of Certified Fraud Examiners (ACFE).
Stopping fraud is an urgent problem, both for the sake of organizations and their customers. Implementing a fraud detection system to reduce losses and mitigate risk brings many benefits, including:
- Preventing losses. By flagging risky behavior, suspicious activity, and other anomalies, a fraud detection system helps stop fraud before it happens - and before losses occur.
- Building customer trust. Preventing fraud losses through robust detection helps ensure customer trust in your organization. Letting fraud slip through the cracks in a way that impacts customers can quickly cause them to take their business elsewhere.
- Compliance with regulations. Organizations - in particular those handling funds and transactions - are required in many places to have fraud prevention and detection measures in place. Failure to do so can result in hefty fines for non-compliance.
- Maintaining operational efficiency. By preventing fraud before it happens, you help ensure that operations within your organization continue as they should - without having to contend with loss of assets, breached systems, or other consequences of fraud.
There are almost countless known fraud schemes that fraud detection systems are up against. Organizations in the financial space are especially vulnerable - and have a duty to protect their customers - so banks and financial institutions must be on the lookout for credit card fraud schemes, synthetic identity fraud, account takeovers, and more. Insurance companies must also be vigilant for fraudulent claims.
Fraud schemes can be broadly categorized into three types: first-party fraud, second-party fraud and third-party fraud:
- First party fraud is when an individual or organization purposely misrepresents their identity or provides incorrect information to gain an unfair or unlawful advantage.
- Second-party fraud is when someone commits fraud using someone else’s credentials, with that person’s knowledge.
- Third-party fraud is when someone’s personal information is used without consent so a third party can illegally or unfairly gain access to resources, credit, or products.
Within these broad categories are many types of financial fraud schemes. Fraud schemes are constantly evolving as innovative fraudsters find new loopholes and opportunities, but there are many types of fraud that can be effectively prevented with strong fraud detection systems, for example:
- Account takeover fraud: Online fraud schemes like phishing attacks are increasingly common. The cyber criminals behind these schemes will impersonate banks via email, text message or phone call to harvest the credentials of their victims. Once they have access to bank accounts, they empty them.
- Credit card skimming fraud: A credit card skimming device copies a credit card when it’s used to pay at a card reader at an ATM, gas pump, or other point of sale. The device owner can then use this information to make fraudulent transactions, such as online purchases.
- Social benefits fraud: Some fraud networks abuse the system of government benefits, such as unemployment. They may take a legitimate unemployment document and tweak it to claim benefits on behalf of multiple persons.
- Mule account fraud: A money mule is someone who transfers or moves illegally acquired money on behalf of others. Criminals use these so-called mule accounts to quickly move their illicit proceeds across different bank accounts and sometimes to foreign jurisdictions, distancing the funds from the true beneficiary.
Read more about common types of fraud schemes.
Organizations often face several challenges in detecting fraud: it is complex, and ever changing. Here’s a look at some of the main challenges around fraud detection:
- Increasing use of generative AI: Criminals are getting creative in their use of generative AI to produce sophisticated phishing messages and deepfakes that appear legitimate. These AI-generated fraud attempts can bypass traditional detection methods by mimicking authentic communications with unprecedented accuracy.
- Evolving fraud schemes: Fraudsters continuously adapt their methods to circumvent existing detection systems. As soon as one vulnerability is addressed, bad actors modify their approach or target new weaknesses, creating a constant cat-and-mouse game between anti-fraud professionals and bad actors.
- False positives: Detection systems frequently flag legitimate transactions as suspicious, inconveniencing customers and requiring resource-intensive manual review. High false positive rates can damage customer relationships and trust while increasing operational costs for organizations.
- Managing huge quantities of data: Fraud detection systems must process enormous volumes of transactions and user interactions in real-time. Organizations often struggle to build infrastructure capable of analyzing this data efficiently while maintaining system performance and identifying meaningful patterns among the noise. Adding to the challenge is data that comes from disparate sources, which can be of varying quality.
- Evolving regulations: Compliance requirements for fraud prevention and data protection constantly change across different jurisdictions. Organizations must continuously update their systems and processes to ensure compliance with new regulations while maintaining effective fraud detection capabilities.
Fraud detection systems are an essential tool for risk prevention teams to catch fraud cases before they get out of hand and cause too much damage. Effective fraud detection also plays an essential role in preventing fraud. The more fraud schemes you detect and understand, the better your defenses, since you can feed that information into risk management models.
Fraud detection systems that fraud prevention teams rely on have evolved considerably over the years. Historically, rules-based detection systems have been at the core of anti-fraud systems. Fraud analysts set up rules to automatically flag suspicious behavior based on known patterns, saving time on detecting common types of fraud, since these systems can process huge amounts of data in a short amount of time.
The downside to rules-based detection solutions is that they often generate a high number of false positives. Rules-based detection solutions are also often poor at detecting sophisticated fraud techniques, complex networks of fraudsters, or previously unknown fraud patterns, leaving gaps in the defenses.
A robust fraud detection system therefore integrates other types of technology to ensure the accurate detection of even the most sophisticated threats. Let’s look at some of the common tools in the fraud detection toolkit.
Transaction monitoring involves real-time surveillance of financial activities to identify suspicious patterns or behaviors that may signal fraud. These systems continuously track transactions across multiple channels, comparing them against established parameters such as transaction amount, frequency, location, and typical customer behavior. When a transaction deviates significantly from established patterns—such as unusually large purchases, transactions from atypical locations, or rapid sequence transactions—the system flags it for further review.
Modern transaction monitoring platforms incorporate risk-scoring mechanisms that evaluate multiple variables simultaneously to determine the likelihood of fraud, allowing organizations to prioritize high-risk activities for immediate investigation.
Data analysis forms the backbone of effective fraud detection by extracting meaningful insights from vast repositories of transactional and customer information. Fraud analysts employ techniques such as anomaly detection to identify outliers in datasets that deviate from normal behavior patterns.
Link analysis helps uncover connections between seemingly unrelated transactions or entities, revealing complex fraud networks that might otherwise remain hidden. Statistical analysis is used to establish baselines for normal activity and identify significant deviations that warrant investigation.
By applying these analytical approaches to historical fraud cases, organizations can identify common attributes of fraudulent activities and develop more effective detection strategies.
Artificial intelligence enables fraud detection systems to learn, adapt, and improve without explicit programming. Machine learning algorithms analyze historical transaction data to identify patterns associated with fraudulent activities, continuously refining their models as new data becomes available. Deep learning systems can detect subtle correlations and complex relationships within data that human analysts might miss. Natural language processing helps systems analyze text communications for fraud indicators, while behavioral biometrics evaluate how users interact with devices to establish unique identity profiles.
Understanding the context around your data is essential for uncovering many fraud schemes, or uncovering them to their full extent. Legacy, rules-based solutions are good at connecting individual data points: a business to an address, a customer to a bank account, etc. But these detection systems are unable to analyze the deeper connections and low-level signals to detect more complex fraudulent behavior or networks of fraudsters.
Graph analytics is a powerful tool for fraud detection. It solves for many of the limitations of rules-based detection systems. Structured as nodes (data points) and edges (the relationships between data points), a graph model enables you to explore not only your data, but also the connections within. This data model is particularly well suited to organize and analyze data where connections are as important as individual data points. Graph analytics can also be used to enhance machine learning models and improve their accuracy.
With an investigation platform like Linkurious, based in graph analytics, you can detect complex fraud patterns that would have been difficult or impossible to uncover otherwise.
Take the example of credit card skimming fraud. A credit card skimming device copies a credit card when it’s slid into a card reader at an ATM, gas pump, or other point of sale. The device owner can then use this information to make fraudulent transactions, such as online purchases. Once a bank receives reports of fraudulent transactions, a graph analytics solution can show if there are common points of sale among the various transactions. These connections can reveal when and where the credit cards were originally stolen. The skimming scheme can then be stopped.
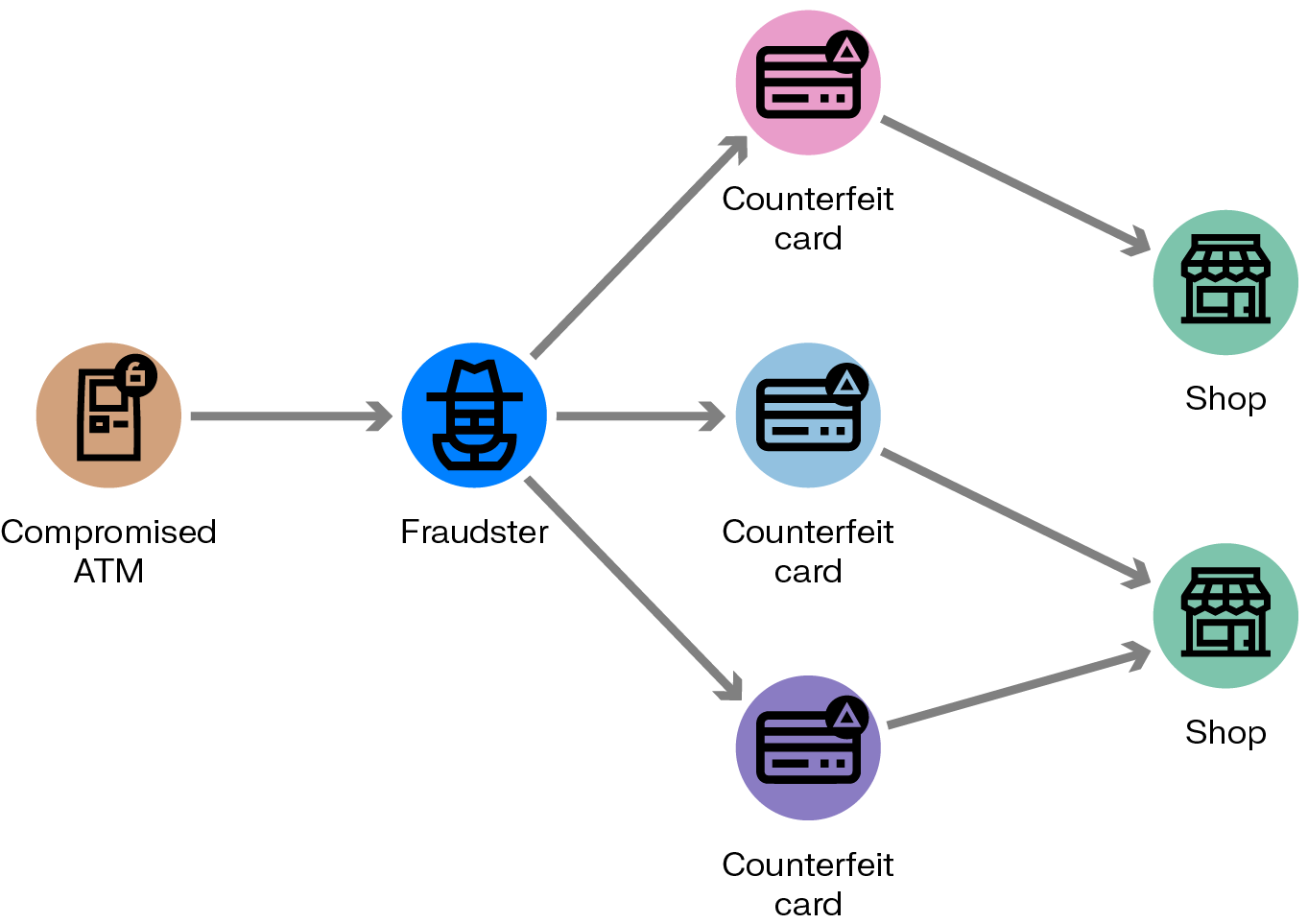
Software solutions rooted in graph technology, like Linkurious, are powerful assets in both detecting and investigating complex fraud schemes. Seamlessly integrating into existing systems, it can help detect more fraud, faster, and accelerate investigation time.
To take a deeper dive into how this technology can improve upon your anti-fraud tech stack, take a look at our e-book: Outsmarting fraudsters with next-generation technology.