Losses due to fraud cost organizations worldwide an estimated 5.1 trillion dollars - more than 80% of the UK’s entire GDP(1). During the COVID-19 pandemic, 93% of anti-fraud professionals anticipated an increase in fraud, with 51% predicting the increase will be significant(2). In this context of increasing fraud, graph analytics are a powerful asset for fraud detection and investigation.
Fraudsters continue to exploit new technology to undermine and target businesses and individuals. In the industry, anti-fraud professionals often describe their fight against fraudulent activity as an arms race(1) that forces businesses and public organizations to constantly find new lines of defense to protect themselves and their stakeholders.
Keeping track of these constantly evolving threats is a particularly onerous problem for business leaders(1). Not only are new threats emerging at an increasing speed, but compliance and risk leaders are under pressure to manage costs and identify, invest in, and implement new technologies to strengthen their anti-fraud arsenal and keep up with increasingly sophisticated fraudsters, who often operate in organized networks.
Fraud detection and graph analytics are a match made in heaven. A lot of fraud use cases require identifying suspicious connections whereas graph analytics is designed to analyze complex connections from big data at scale. In this article we will provide a series of examples where graphs can be used to fight back against sophisticated fraud schemes.
The problem: When criminals want to defraud a bank or launder money, they typically try to cover their tracks. One approach is to create fake identities to commit their wrongdoings. A synthetic identity is a fake identity mixing real or fake information (such as a real social security number or a fake name) that does not belong to a single real person. Detecting synthetic identities can help stop criminals before they commit financial crime.
How graph analytics can help: Graph-based technology can help detect rings of clients interconnected across personally identifiable information such as an address, a phone number, a date of birth, an IP address, etc.
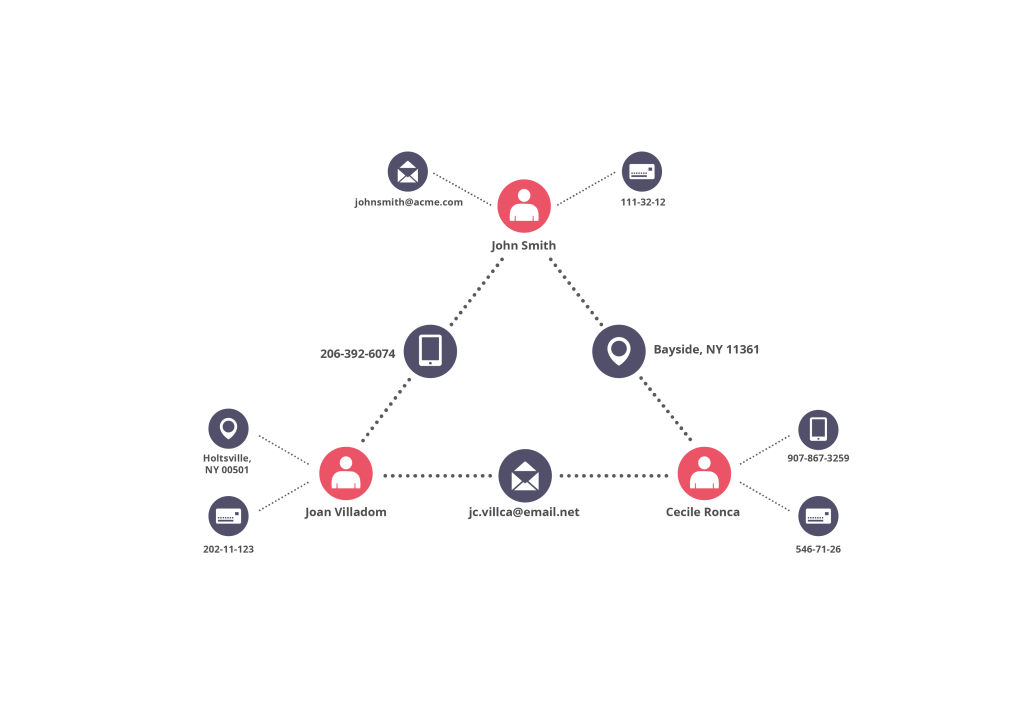
The problem: In an ideal world, each individual or company in your databases would be unique. Chances are though that it’s not the case. Maybe the John Smith that exists in the retail bank database has a different ID than the John Smith of the consumer credit database or of the John Smith in the company owners database that you bought, despite the fact that these 3 individuals are actually the same person. Avoiding this sort of duplication is a complex problem in an IT system with different silos.
How graph analytics can help: Graph can detect common links across different entities to help identify potential duplicates. Maybe even though our 3 John Smiths have different IDs they all share the same date of birth, the same address and the same phone number. If so, chances are that they are indeed the same person.
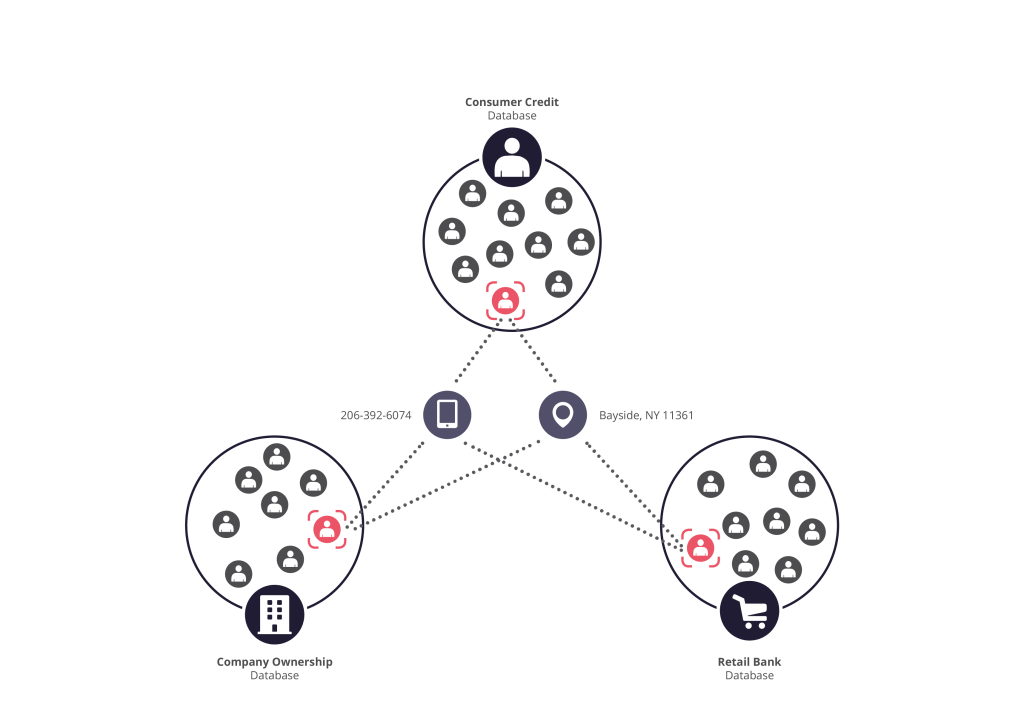
The problem: Sometimes a tip or a fraud detection system may flag a client or behavior as suspicious. A critical thing to assess in this case is whether this single suspicious situation is isolated or not. The client may be part of a larger criminal ring or the behavior may be part of a bigger scheme. Without more information, it’s important to explore as many leads as possible. This requires exploring what the client or behavior are connected to. This can be a very cumbersome process when the data is heterogeneous and scattered across different tabs. Who is a person connected to directly and indirectly via financial transactions? This requires opening a first tab with the person’s transactions and their recipients. Then you’d need to open the same sort of tab for each recipient.
How graph analytics can help: Graph analytics facilitates the dynamic exploration of relationships within a large dataset. It’s possible to explore and visualize who and what a client is connected to across information as diverse as an address, a phone number, an email, transactions, etc. Detecting accomplices becomes faster.
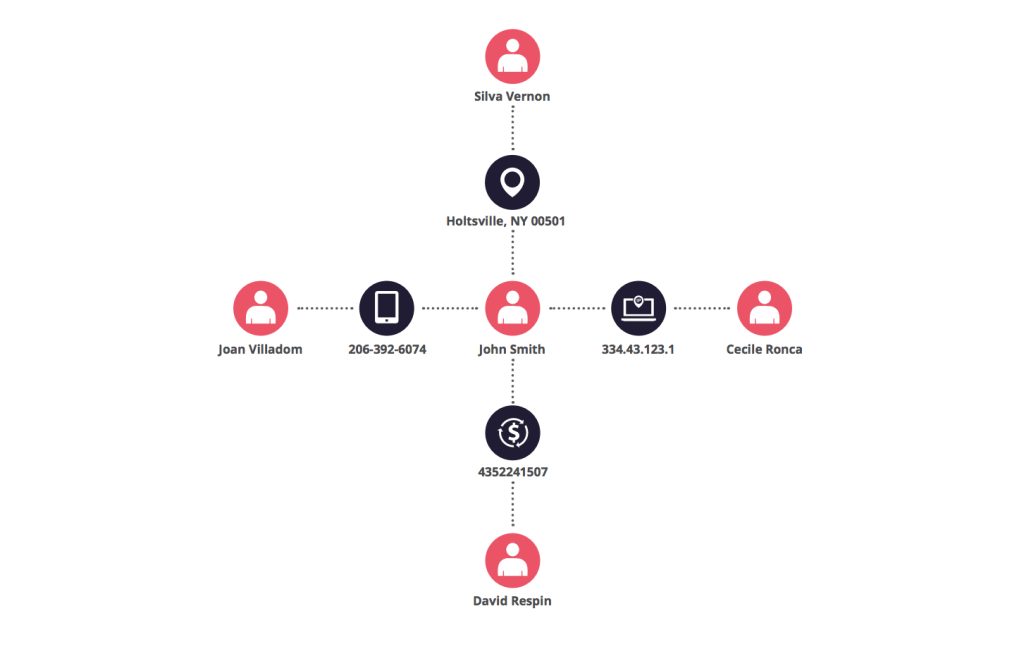
The problem: Some fraud networks abuse unemployment benefits systems. It’s possible for example to use a legitimate employment document and tweak it to claim unemployment benefits on behalf of multiple persons. The persons involved may keep a percentage of the stolen money and share the rest with the scheme’s organizers.
How graph analytics can help: Since the scheme revolves around reusing legitimate documents to create multiple fake ones, links between the perpetrators naturally occur. The fraudsters may be connected to the same company and oftentimes are interconnected across phone numbers, addresses, bank accounts or IP addresses.
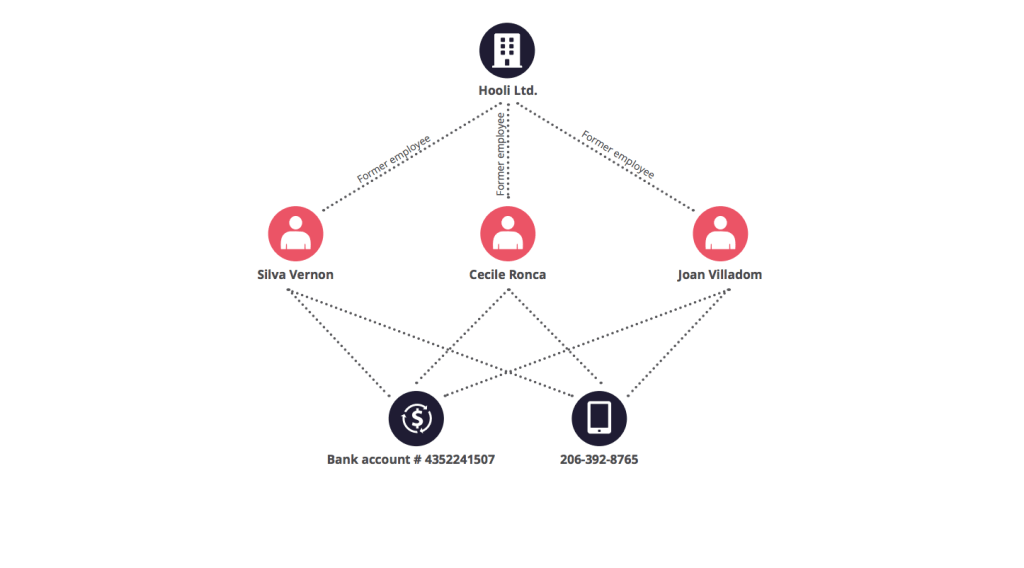
The problem: By arranging a series of international transactions between cooperating companies, it’s possible to claim a VAT refund without ever paying the VAT in the first place. It’s estimated that VAT fraud costs £170bn per year in the European Union.
How graph analytics can help: Operating the scheme requires a company that can charge the VAT tax to a client and then disappear without ever transferring the money to the tax authority. Fraudsters thus need to take over or create multiple companies. Oftentimes these companies end up sharing pieces of information: a registry address, a director, a contact number, etc. If a company disappears without paying the VAT tax, graph analytics can be used to identify other potentially suspicious companies to react before further damage occurs.
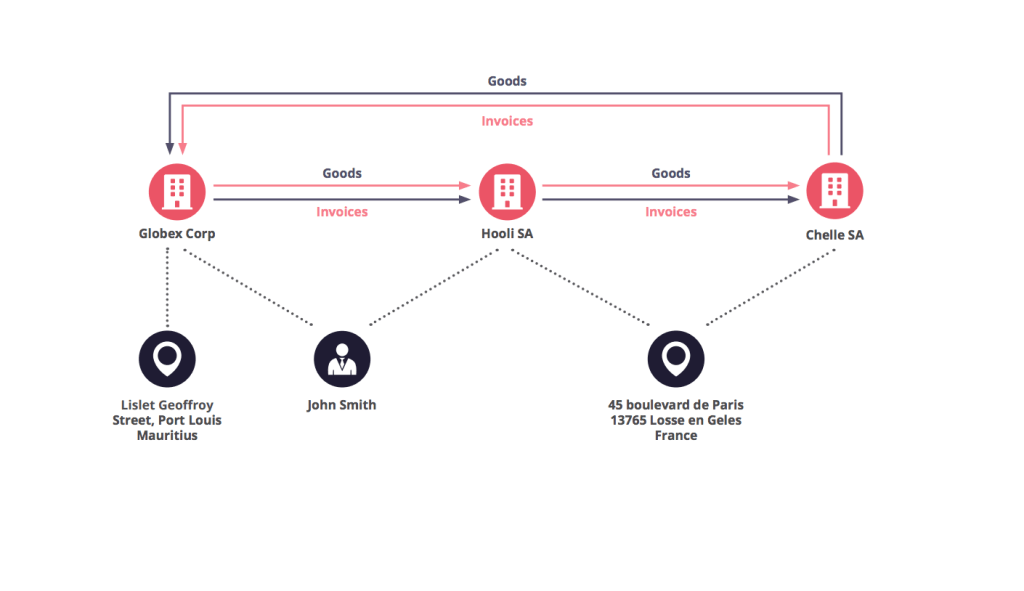
The problem: Corrupt practitioners can bill for services they haven’t provided. Institutions such as Medicare pay those bills. It’s particularly hard to stop this when the practitioners claim those services were performed on older patients.
How graph analytics can help: Fraud graphs can help spot anomalies such as patients seeing multiple doctors for the same procedures, practitioners sharing many common patients or doctors billing the same services multiple times to the same patient.
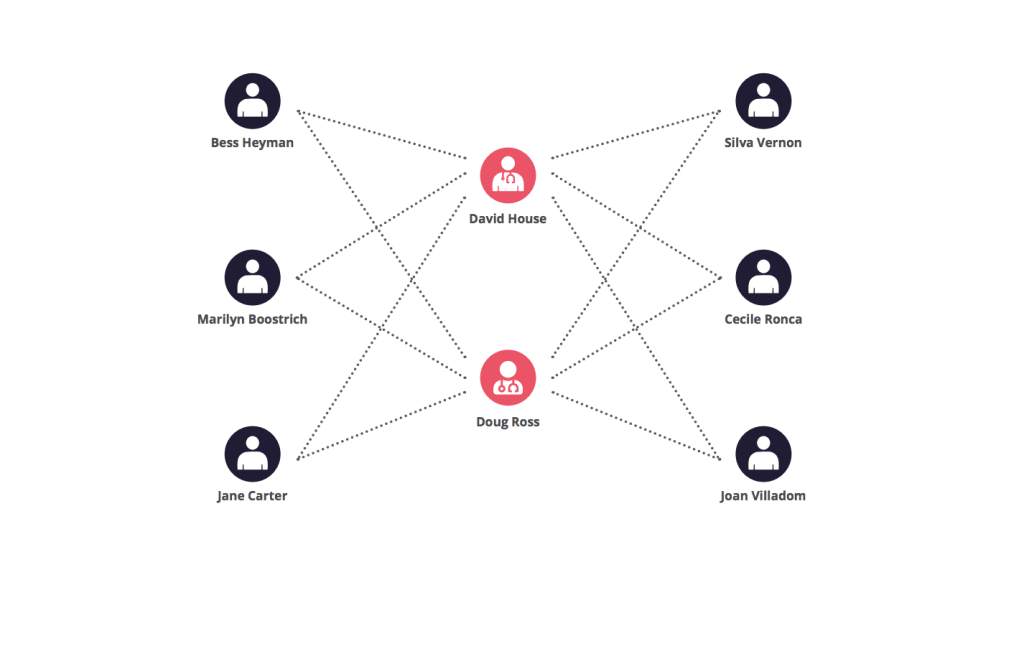
The problem: Generating fake insurance claims can be a way for fraudsters to make money. An individual can take a car insurance policy, then claim a refund on a non existing car accident with the help of a car repair shop.
How graph analytics can help: Scaling fake car accidents requires multiple policyholders, multiple cars and multiple car passengers. Graph analytics can help identify if within an insurance company’s client database multiple people are interconnected across cars, individuals, repair shops or claims. The larger the network, the more likely that fraud is going on.
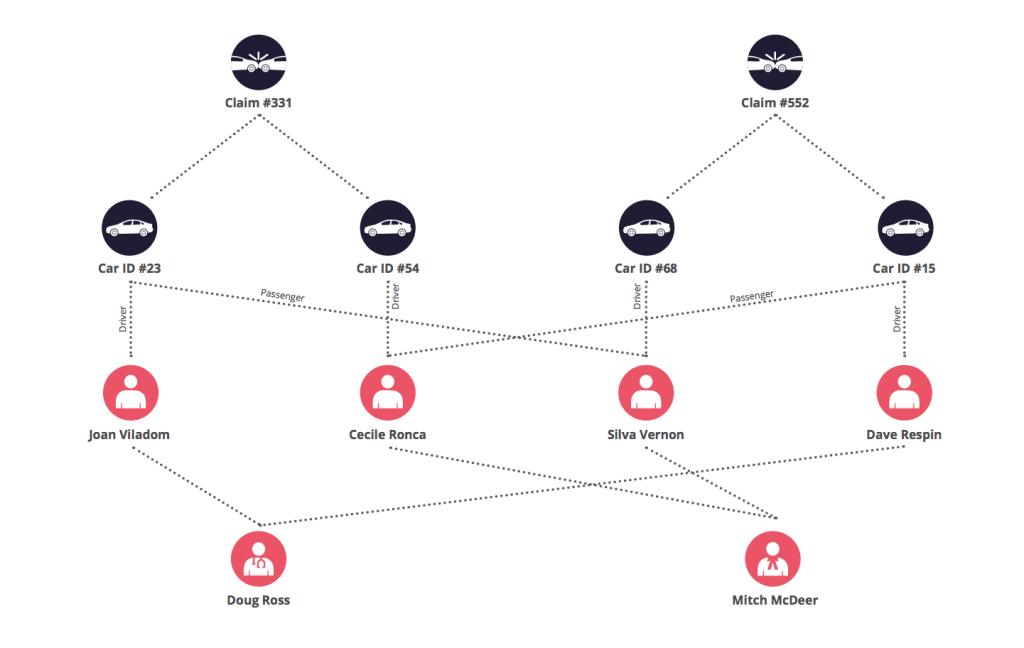
The problem: A credit card skimming device copies a credit card when it’s slid into a card reader at an ATM, gas pump or other point of sale. The device owedner can then use this information to make fraudulent transactions (e.g. make a purchase online).
How graph analytics can help: The fraudulent transactions enabled by the skimming device are eventually reported as such by the cards owner. Are there common points of sale among the various transactions reported as fraudulent? These connections can reveal when and where the credit cards were originally stolen. The skimming scheme can then be stopped.
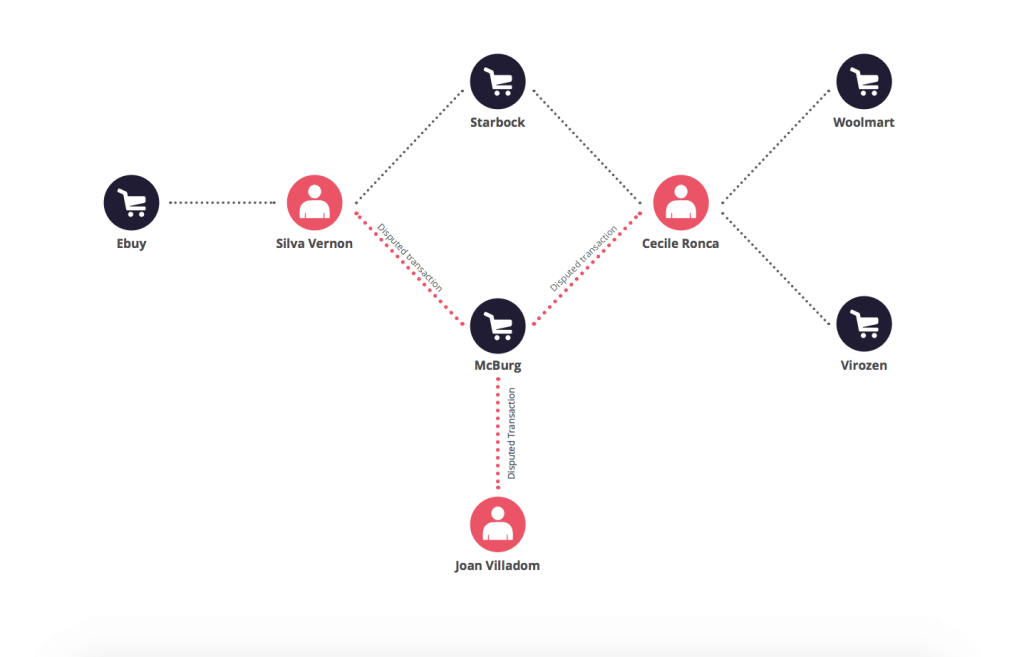
The problem: Referral programs incentivize individuals to promote a product or company to their personal networks (eg “win $200 if a friend creates an account”). These programs can be targeted by fraudsters who act as a group to generate referrals in order to capture the monetary rewards. Meanwhile, the company is not generating any business out of these fake clients.
How graph analytics can help: In referral programs, a client refers other people as potential clients. What if some people are tied via personal information (IP address, bank account, address) to other people within the network or to other networks of referrers? Graph analytics can help identify these connections and thus uncover entire networks of referral abusers.
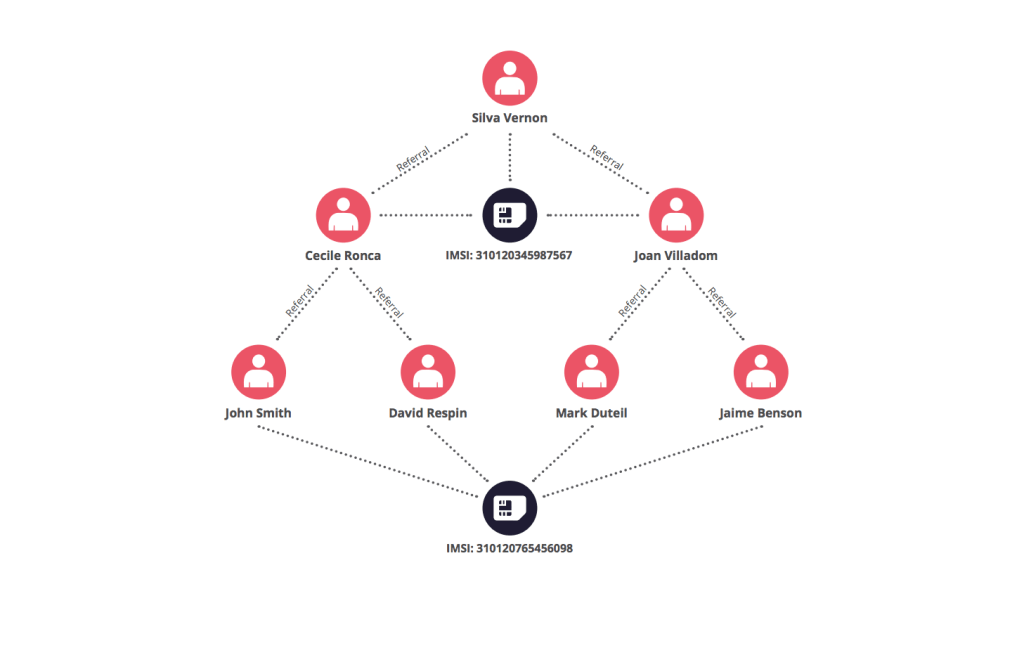
The problem: Employees handling credit loans can defraud the bank they work for. Accomplices can ask the bank for loans (using real or fake information) that the employee then accepts. The money can then be shared among the scheme’s perpetrators who never pay back the bank.
How graph analytics can help: Is a given employee tied to multiple bad loans? Are there connections among clients with loans? Is there information within the loans shared across multiple loan applications? Graph analytics can help uncover those similarities and expose fraudsters.
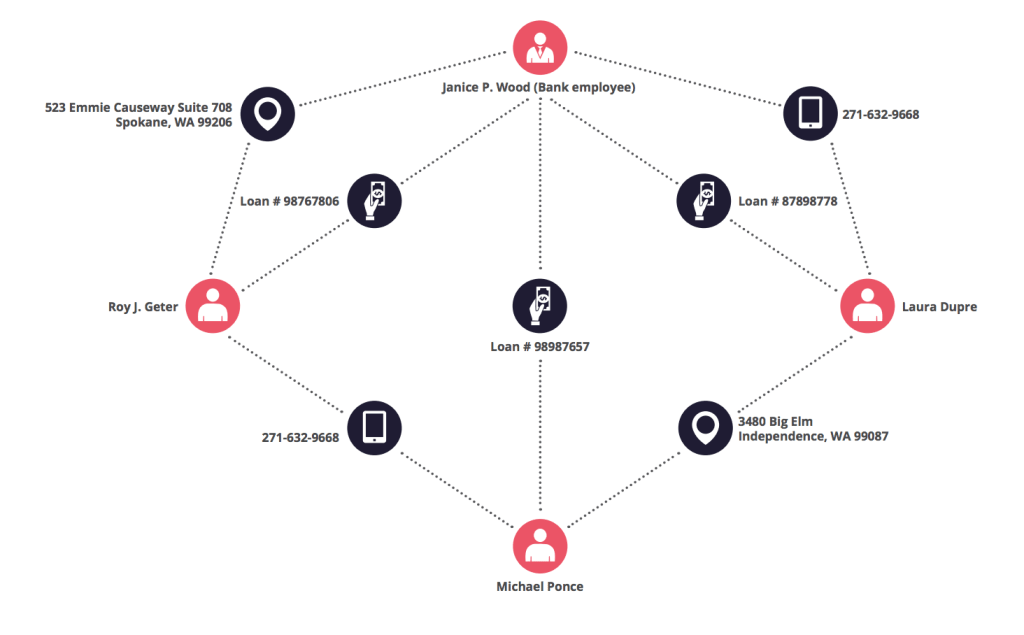
The problem: Any company spends money to operate, giving an opportunity to fraud networks to take advantage of weak controls in procurement processes to fly under the radar among numerous procurement activities. What if a rogue employee coordinates with a supplier to defraud the company? There’s potential for substantial money losses.
How graph analytics can help: If the employee has ties with the supplier such as a shared address or actually controls the supplier indirectly, fraud graph analytics can help identify such connections.
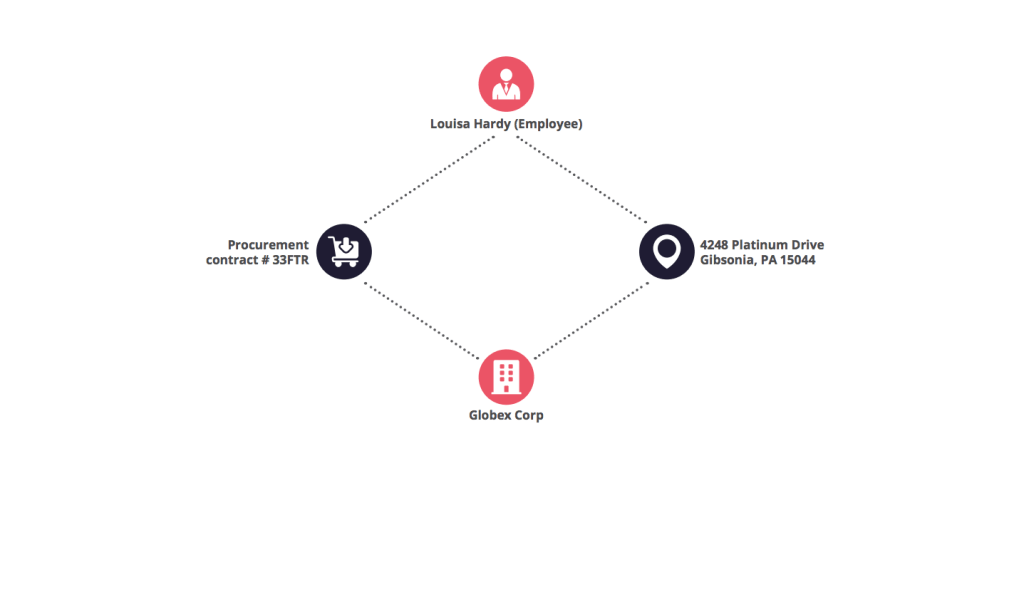
The problem: Public tenders are meant to enable an open competition to ensure low prices. What if the competition is rigged? Maybe a corrupt employee is cooperating with criminals to syphon off money. In this case, multiple organizations may take part in the tender to give the impression of competition but may actually collude to charge a higher price.
How graph analytics can help: The companies taking part in the public tender may be connected via capital links, common directors or intermediate shareholders. Graph analytics can reveal those links and uncover the corruption.
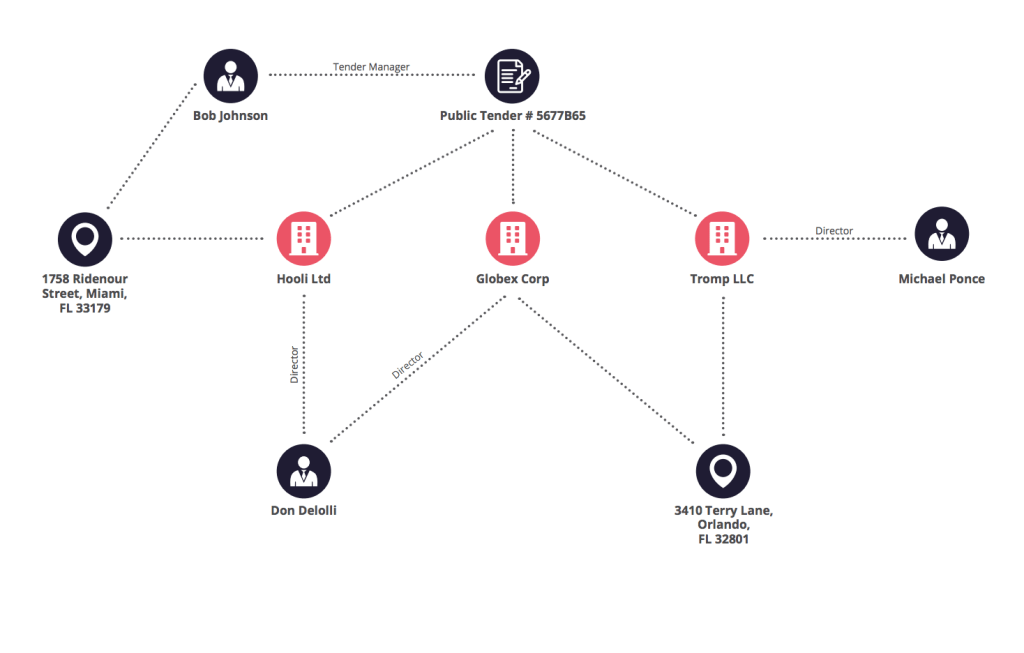
The problem: Many countries set up programs such as the Paycheck Protection Program (PPP) to provide funds to companies impacted by COVID 19. Such programs offered an opportunity for fraudsters who use forged documents to send multiple fraudulent applications to the PPP and receive funds.
How graph analytics can help: The forged applications often have common information. The IP address used to fill the applications can be the same. The company’s owner, the bank accounts, the addresses can also be shared. Graph analytics can help identify those identical information and unmask the criminals.
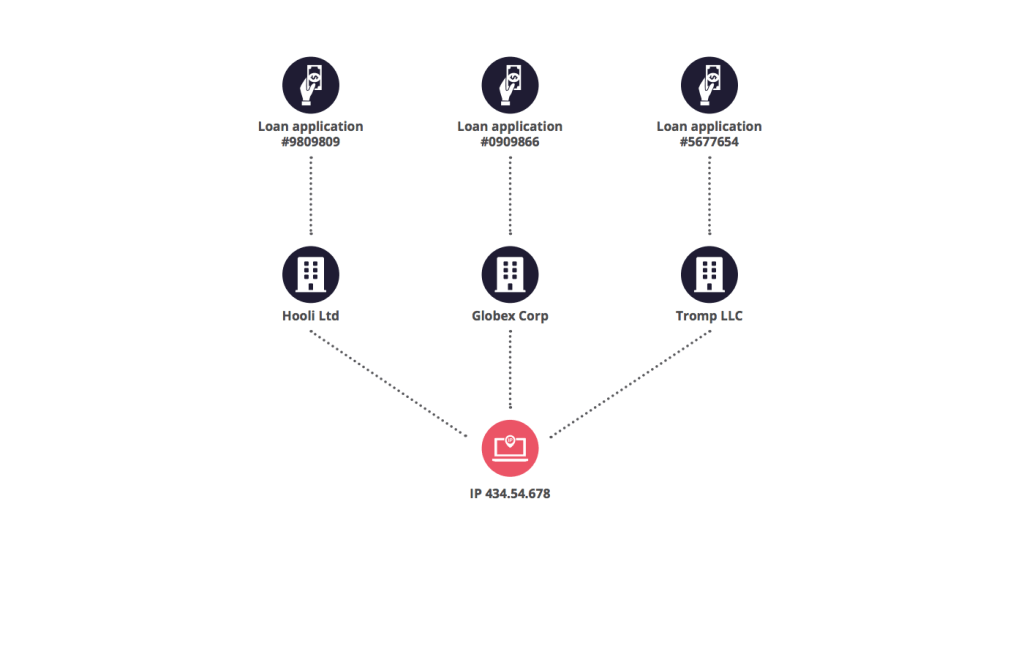
The problem: Some cyber criminals send emails impersonating banks to harvest the credentials of their targets. This approach is called phishing and allows criminals to access their victims’ bank accounts and empty them. This represents a major threat for financial institutions in terms of brand equity and can severely damage the relationship with their customers.
How graph analytics can help: When a phishing attack occurs, there’s usually more than one account which is compromised. Graph analytics can help spot a new IP address being used to access multiple user accounts or a new bank account which is added as a recipient of funds by multiple user accounts.
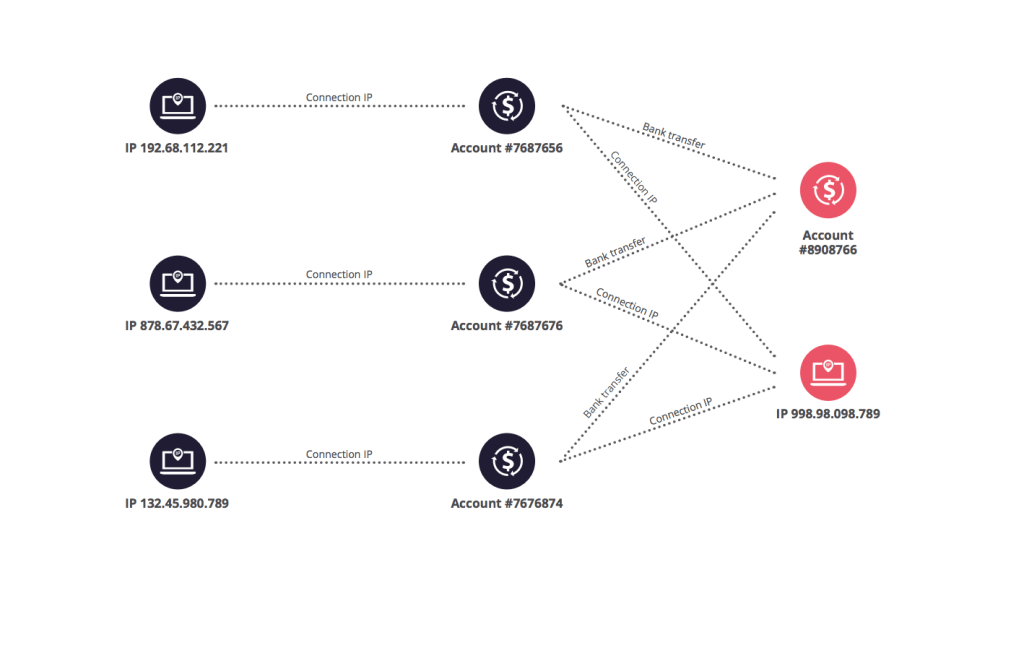
The scenarios in which graph analytics can be beneficial in helping to detect fraud cases continues to grow. As criminals become more and more sophisticated in how they abuse financial institutions or tax agencies, so too do the tools that help fight back against them. White collar criminals don’t stand a chance when anti-fraud teams are equipped with graph analytics.
Download our ebook on outsmarting fraudsters with next-generation technology and learn more about where current anti-fraud systems fall short and how next-generation analytics can help stop fraudsters in their tracks.
A spotlight on graph technology directly in your inbox.