The pillars of knowledge management: Interview with Sara Duane of Enterprise Knowledge
With ever growing sources of data, content, and knowledge, what matters for organizations today isn’t just what information you have, but also how you can manage and use it. Knowledge management has emerged as a valuable discipline that gives organizations the capacity to optimize their use of data and information flows.
We wanted to take a deep dive into knowledge management, so we sat down with an expert: Sara Duane, Senior Consultant at Enterprise Knowledge, a consulting firm specializing in knowledge, information, and data management - and a Linkurious partner. During the interview, Sara spoke about the essential pillars of a solid knowledge management plan, the technology behind knowledge management, how the domain is evolving, and more.
Could you start by introducing who you are, what your role is and a little bit about Enterprise Knowledge?
My name is Sara Duane. I'm a Senior Consultant at Enterprise Knowledge.
Enterprise Knowledge is the world's largest dedicated knowledge, information, and data management consultancy. We have over 80 consultants dedicated to the knowledge management domain who support strategy, design, and implementation. We work within multiple sectors, specifically in thinking through how we can plan for organizations’ knowledge management strategies. We do a lot of work within the semantic engineering space, thinking through how we can better optimize organizations’ data and information flows, as well as content management practices, and enabling enterprise AI.
We answer questions like: Can users find the right information at the right time? Do data sources have clear context for users to know what information they contain? Can users discover relevant data so that they use everything possible to inform their work?
Within all that, I sit within our strategic consulting practice area as well as the semantic consulting practice area. I specialize in helping our clients prepare for the buy-in and the continuation of their knowledge management strategies and plans. I do that through creating the roadmaps and the strategic definition for knowledge management.
I also help implement the components that make up that plan. That might be implementing a knowledge graph for use in a recommendation engine or a chatbot or semantic search, depending on the knowledge management plan.
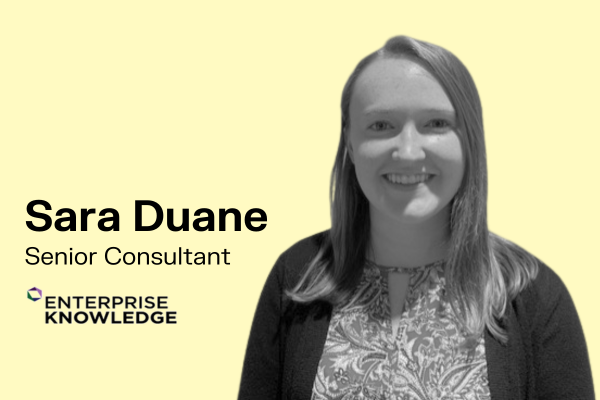
What kinds of organizations is Enterprise Knowledge working with? Do you focus on certain types of sectors or is the work pretty broad?
The great thing about knowledge management is that every single person, whether they've heard of knowledge management or not, has been impacted by it. It’s really for this reason that we work across sectors.
Knowledge management looks at how you structure, find, and organize your information. We work with a range of clients, from nonprofits to insurance and investment companies to pharma to the federal government to educational institutions. Recently, though, we've been seeing a big emphasis in finance and pharma specifically, especially within our semantic layer work.
It's interesting, because the business value and the business case is so different depending on what industry you're in. When we talk to a non-profit thinking about knowledge management, for example, at the end of the day, their goal is to more effectively use the donor dollars that are being provided to them. Whereas, for a financial organization, it might be, how do we increase effectiveness in order to increase the number of features we can push out in a two week span and increase revenue.
Digging more into knowledge management itself, I want to ask you what knowledge management is exactly: how would you define it? And why is it important for so many types of organizations today?
At Enterprise Knowledge, we like to describe knowledge management as involving the people, the processes, the content, the culture and the technology that is really necessary to capturing, managing, storing, sharing, and finding information and knowledge within an organization.
Like I said, it really impacts every industry because everyone needs information and knowledge to do their jobs, no matter where they are. But the focus of that definition, and what makes it really important, are those verbs. The capturing, the managing, the sharing, the finding information are the actions we spend our days on.
The goal of knowledge management is to make those actions easier. The way to tackle that, and the framework for how we do it at Enterprise Knowledge, is through those five pillars: people, process, content, culture, and technology.
- People is all about how the information may flow from person to person or department to department within an organization. It's about the people who have the knowledge and the people who need it. And at the core of that are the experts in specific subject areas or organizational knowledge.
- Process is about the kind of governance and consistency of governance within an organization. How clear are the processes to go from the beginning of your operational flow to the end? Are they followed? Are they clear?
- Content and data. Within this framework, this is really about the state of the content and data. Is it high quality? Is it clear? Are there a lot of duplicates across the organization? How dynamic is it?
- Culture is about the culture of knowledge management and organization. Are people willing to share? Are they willing to collaborate to innovate? Do they have leadership and senior buy in and support?
- And finally, technology. We purposely list it last because it's obviously a very important part of knowledge management, but we like to think of it as that enabling factor. Technology is what makes up the knowledge management system. It’s an important piece of the puzzle, but you need those other four framework pillars in place in order to make the technology useful and helpful.
One of the challenges knowledge management is responding to is the exponential increases of content and data across organizations, especially with the rise of generative AI. How do we make use of it? How do we monitor it so that it's both governed well and then leveraged for all the insights that are within it? How do we make sure there aren’t silos within the organization? Knowledge management is at the core of a lot of those challenges.
It also improves things like content findability and decreased content loss, but it also gets at those key business value challenges as well.
Think about a call center. To me, this is an understandable example of knowledge management. In this context, it's not just about making an organization’s content more accessible for their call center representatives. The business impact of that improved KM is the number of calls they can take within the hour and the customer service satisfaction that comes from it. The agent is able to find the resources they need to give someone the right answer, and the larger operations of that department work a lot smoother.
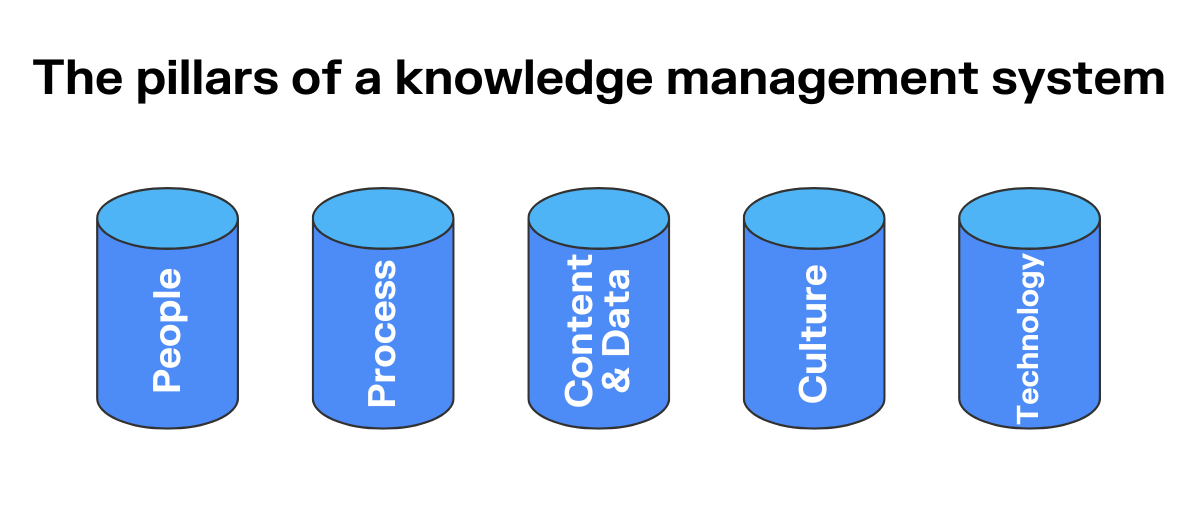
Because Linkurious is a technology company I did want to dig into the technology component. Could you talk about the kinds of technologies that typically power a knowledge management system?
Of course. Just because technology is enabling, it doesn't mean it's not important! It's a big part of our work at Enterprise Knowledge. When you think about a knowledge management system, the technology that powers these information flows - there's a lot that goes into that.
First, there are the key repositories or data sources where data is living. For an organization, that might be a document management system or a CRM. Or, back to that call center use case, there might be a ticketing system.
Then, there is the findability and connectivity layer sitting on top of that. We'll talk more about the semantic layer throughout this discussion, but the findability layer is really aligned to what the semantic layer is trying to get at. It’s creating a level of abstraction, which means a layer of findability for that content, no matter where it sits within a specific data source. That happens through things like enterprise search, a knowledge graph, through ontology or taxonomy that allow us to give structure to our data models and classify the way we're thinking about the relationships and the definitions of terms.
It might be things like a chatbot or a recommendation engine. Those are often for more advanced use cases, but we've seen a lot of organizations go those routes recently.
There is also reporting that's happening, creating insights around things like usage metrics. Meanwhile, you also have background operations going on, like auto tagging and auto categorization of content.
There is the collaboration layer. This might be through things like Slack or Teams or SharePoint.
Finally, there are the pieces that connect the whole system, through APIs and through the ETL processes that flow between them.
Yes. In a given organization, they may have all of those pieces or they may not. A lot of organizations come to us knowing a specific need, say, for enterprise search. From there, they're building out their semantic layer and that's their starting space. I didn't touch on data catalogs, but that's another example of the findability layer leveraging metadata. An organization might start there and then we'll build from that point. There are use cases for each of those components. And an organization may not have them all.
You've touched on it a bit already, but what are some of the most compelling use cases you see for knowledge management?
There are a few examples of use cases I can give that I think make sense to people who might not have heard of knowledge management.
The first is “softer” knowledge management, one that is less technology focused. It's things like communities of practice. Within an organization, there are lots of people who have areas of specialization and interest, who may want to create communities of people interested in similar project types or similar growth areas for example.
Creating a community that's very invested in knowledge sharing and knowledge transfer in a specific topic area is an important application of knowledge management. It helps address some of the knowledge loss that happens when people leave an organization, transfer departments, or retire. There are many ways to bring technology and innovation into that as well–thinking through how we can use AI to facilitate capturing tacit knowledge and making it more usable for a community of practice.
There are also applications that help deliver insights and information to people at their time of need. Things like content personalization or an expert-finder use case. It lets you find the right expert in a specific area when you need them and to know who to reach out to, based on who in the organization has worked on similar projects before.
Content personalization is similar. When you're working at an organization and you're searching for a how-to article, or maybe your standard operating procedure, and you just get back a list of documents, it may not be that helpful. You find your document, but you might actually be interested in bigger questions like, how does this document relate to the larger process that I'm working in? How does this document relate to specific people who could help me answer it? That’s where the technology piece of the semantic layer comes in, making those connections between core concepts at an organization clear and accessible for people when they need it.
Those are just a few examples. There are plenty more: enterprise or product 360, etc.
Since Linkurious is an Enterprise Knowledge graph partner, I’d love for you to talk a little bit about the role that graph technology plays in knowledge management. In what way does it add value to the tech component of knowledge management?
Graph is often a huge part of the way a lot of organizations seek to tackle their knowledge management challenges, for a lot of reasons. But the main way we see graph integrated into organizations is as a component of the semantic layer.
The semantic layer is that layer of abstraction for findability. It's the relationships. It's the domain knowledge. It's the context behind data at an organization that's pulled out from key data sources.
The organizations we work with have a lot of diverse data sitting in many different sources. It's often not being unified or used to its full potential. Graph technology comes into play here, providing context and an understanding of relationships between information within a domain. It shows how the pieces fit together. It also allows us to store information in both a human understandable way and a machine understandable way so that you're able to leverage the graph to answer questions in the way humans may ask them.
Let’s think back to the expert-finder example: who in my organization has worked with a specific client? That’s a way you and I would ask that question. And graph allows us to translate the way a human would ask that question into the way machines understand that question. This is the way a graph stores the nodes and the information within it.
We see a lot of organizations using graph for both structured and unstructured information - things like large notes documents, large reports that are stored in people's shared drives - while also using the structured information that comes out of large data reports. It all fits within the graph and gives you the ability to think through that aggregation at a higher level.
At the end of the day, it connects back to the knowledge management needs that we were talking about at the beginning. Graph solves a lot of those KM challenges. It makes content more understandable and gives us the ability to push content to people when they need it. Answers are being shown to them through the concept of discoverability.
In terms of the graph visualization component, is that something that can be used to enhance user experience? You were talking a little bit about connecting the human and machine and for us Linkurious, we have a software that's intended to be accessible to all types of users, whether they're technical or non technical. We want to make data accessible to everyone. I'm curious how graph visualization comes into play within the knowledge management context.
As I mentioned, graph brings so much value to organizations. The data model describes the domain or the industry that an organization sits in as well as the content and information about that organization specifically.
There's a lot you can do with that. Visualizing all of the information in a graph helps you use and manipulate the graph in a way that makes it more understandable for an end user. They can then make jumps and inferences themselves.
In fraud detection use cases, for example, end users can see where connections might be across claims and a potential fraud instance. Graph visualization shows them how those potential situations or instances are related using the information that's stored in the graph.
This is especially helpful for someone who doesn't want to see all the technical elements behind the curtain. They want to see the human understandable knowledge that a graph gives you, which is all about those relationships. Visualization also lets us address use cases like personalization by delivering those reasoning and inference pieces.
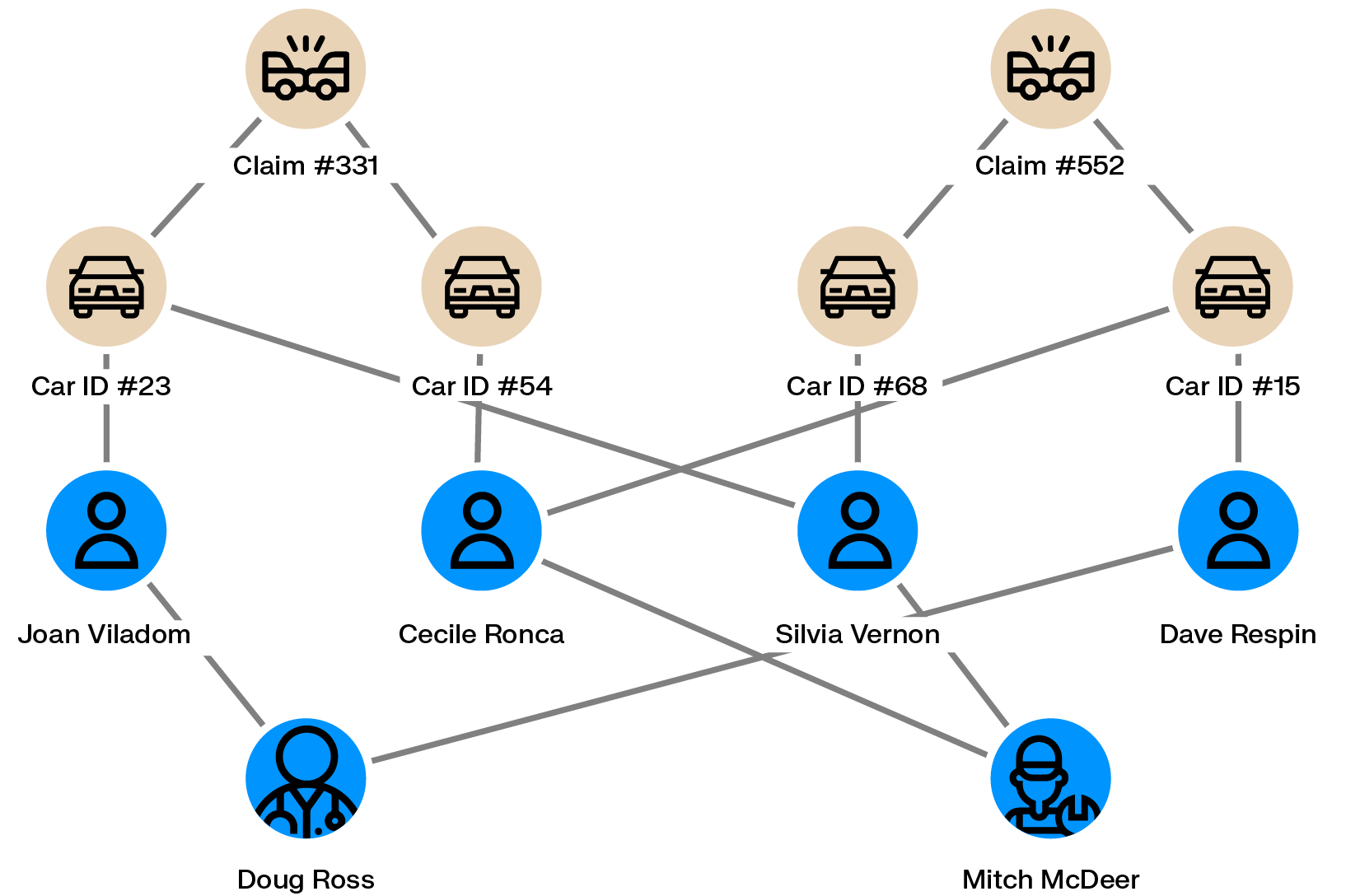
I was wondering if you would say that knowledge management has a role to play in decision making for organizations, and in what way?
It definitely does. At the core of knowledge management, one of our “basic” goals is facilitating discovery of content through content standardization and making sure it’s high quality. But as we get into more advanced applications, KM use cases move towards things like creating opportunities for advanced reporting on your content and data, creating the ability to make insightful, effective decisions based on content and data that is up to date and correctly organized.
Effective knowledge management also provides value beyond decision making. It helps in the identification of risks and opportunities, the prediction of what's going to be the next thing for your organization, or the next key challenge that you'll need to address.
From a solution standpoint, for a use case of decision making, something like a chat bot or a recommendation engine or an expertise locator may come in. Using graph for those solutions makes them all the more contextualized and all the more domain specific.
I wanted to finish up by asking you a couple of broader questions about knowledge management. First, are there emerging trends in knowledge management that you find particularly interesting or exciting?
Definitely. With the kind of push and excitement around gen AI over the last year or so, we're seeing two main trends.
First - and this is really relevant to us as partners - is the push towards using graphs and using the semantic layer for that domain context for some AI-based solutions. We’ve been working with graphs since 2017 or 2018, so it's exciting to see that people are understanding that you can't just throw a “black box” AI solution at something. It’s more valuable and insight driven when you add something like a graph to a given solution to provide that domain context.
It makes it so the more advanced solutions are not so black box. A lot of solutions can give deterministic reasons for why one piece of content is associated with another, or why someone's getting the answer they are, because we can connect back to the rules and the triples within our graph.
Another theme I’m seeing is that, because of the push towards gen AI, on the other end of things, people are also more and more interested in the people, the process, the culture, and the governance aspects of these things. It’s exciting to see because, while technology is very important, you need the frameworks in place in order to make it helpful.
We see people asking the right questions around their organizational frameworks. Do people have good onboarding processes? Do they know where to find the information they need when they start at a company? Are you making sure that your organization is taking the knowledge from people who are leaving and making sure that's integrated within your larger information structure?
In this vein, we’re seeing a push towards some of our softer KM initiatives as well, but with a technical flavor to them. Things like, understanding how we can use advanced AI solutions to help make tasks like knowledge transfer from someone who's leaving an organization easier.
To conclude, what advice would you give to organizations who are looking to either put in place a knowledge management system or improve their knowledge management practices?
My biggest recommendation is that organizations focus on all of those five pillars of knowledge management: people, process, content, culture, and technology.
You can't just throw technology at a problem and then leave. You need to think of technology as enablement. It's enabling the people to find the information they need. It's enabling the processes in place. It's creating a culture of innovation and sharing.
The other piece of advice I have is about making a strong business case for knowledge management, not just through the KM outcomes that you're getting, but also through the business outcomes and business value. So, yes, it’s easier to find content, and yes, my content is not duplicated. But you also need to connect it to business value.
Let’s think about that call center example again. Part of the value generated for the business is that they’re able to help more people within an hour. Really emphasizing the things that tie into a business’ strategic goals is what really helps push knowledge management at an organization.
At the end of the day, your leaders will be happy that it's easier for their company, their staff to find documents and answers, but they care about the end application and what it does for the business.
And finally, I would say it’s important to start small and pilot changes within those five pillars.
That means you might pilot the start of a community of practice or do a proof of concept of a graph, and then create a visualization that folks are able to use and try out and leverage, building from there.
So starting small, iterating, and adopting changes as new things come up is really key.
That’s also where we at Enterprise Knowledge come in. We'll meet with organizations, look at the big picture, and help them implement a KM plan. We help with creating that target vision for where an organization wants to go with KM as well as the plan to get there. We also work on the implementation of those pilots. We get to tackle knowledge management from all sides and that makes it really interesting.
A spotlight on graph technology directly in your inbox.