Reducing AML false positives with graph and AI technology
To stay compliant and to prevent money laundering from occurring, financial institutions must be well aware of the activity and transactions going on within their organizations. They also need to be able to report any suspicious transactions in a timely manner to their local FIU. To ensure they’re able to meet these needs, virtually all financial institutions are working with AML screening and monitoring systems to flag suspicious activity for further investigation.
But anyone working in the compliance field within the financial services sector knows that, while legacy systems serve an indispensable role in monitoring for suspicious activity, they can also leave some holes in the net while also generating huge volumes of AML false positives - not to mention costly false negatives. That’s where AI and graph technology can step in.
This article looks at some of the main challenges for AML analysts and investigators, and why we need more efficient AML systems today. Then we’ll look at how, by adding graph analytics and visualization and AI through tools like Linkurious Enterprise and Dataiku, compliance teams can easily enhance existing setups to improve efficiency and reduce the number of AML false positives.
Anti-money laundering systems are working - but not efficiently enough
Certain factors are pushing regulated organizations to review their anti-money laundering approaches today.
First, banks are seeing the limitations of their legacy rules-based approaches. They drive significant volumes of false positives - up to 95% in some cases (1).
The second factor is cost. With legacy systems churning out high levels of false positives, financial institutions have to find a way to work around this. For many, the solution has been increased human intervention on AML alerts to sort out the false positives from the cases that merit further investigation - but this is expensive.
Finally, there is increased scrutiny from regulators globally, resulting in increased fines. In 2022, money laundering fines increased 50% compared to the previous year (2). This has acted as an incentive for financial institutions to improve their controls.
AML tools that don’t quite cut it
Within the market tools financial institutions are using to tackle money laundering, there are also multiple challenges.
Many of them allow for only a low level of customization, making it difficult to adjust tools to evolving criminal threats. They are also often unable to use complex models for monitoring, detection and decision-making. And, as we alluded to above, traditional pass/fail controls result in a significant percentage of false positives. By focusing on individual data points and simple rules, traditional systems often miss key connections and the broader context that may be indicative of suspicious activity. They can also miss complex criminal schemes.
Combining AI and graph technology for enhanced AML
In most cases, financial institutions have invested a lot of time and resources in their existing systems, often over the course of many years. Ditching these systems for something new therefore may not be a viable option. The good news is that it’s entirely possible to enhance existing legacy systems with newer technology, such as graph analytics and visualization, and artificial intelligence.
Two such tools that together can help enhance traditional AML systems by working alongside them are Linkurious Enterprise and Dataiku.
These graph and machine learning tools can be rolled into a single solution for investigators, providing significantly better efficiency. They do this by leveraging graph analytics and machine learning to generate more relevant alerts by reinforcing existing systems and eventually replacing them. Graph visualization capabilities accelerate and improve the quality of investigations. And these solutions create an efficient, agile back-testing environment that enables you to define appropriate indicators and scoring approaches to effectively reduce both AML false positives and false negatives.
Reducing AML false positives with machine learning and graph
As an example, we’re going to look at how Linkurious Enterprise and Dataiku can enhance transaction monitoring to reduce false positives. Linkurious Enterprise is an off-the-shelf data visualization and analytics software that leverages graph analytics to enhance each step of the investigation process. Dataiku offers a complete, end-to-end data platform for analysis, machine learning, and more. It lets users develop, deploy, and operate analytics at scale.
In this example, you can set up machine learning rules in Dataiku alongside your traditional business rules, and then act on the alerts they generate directly in Linkurious Enterprise.
In this setup, Dataiku is used to manage the underlying graph database itself and ensure it has the information and is well managed. Linkurious Enterprise is available to ensure that your interaction with your graph and your discovery process within it is of the highest caliber.
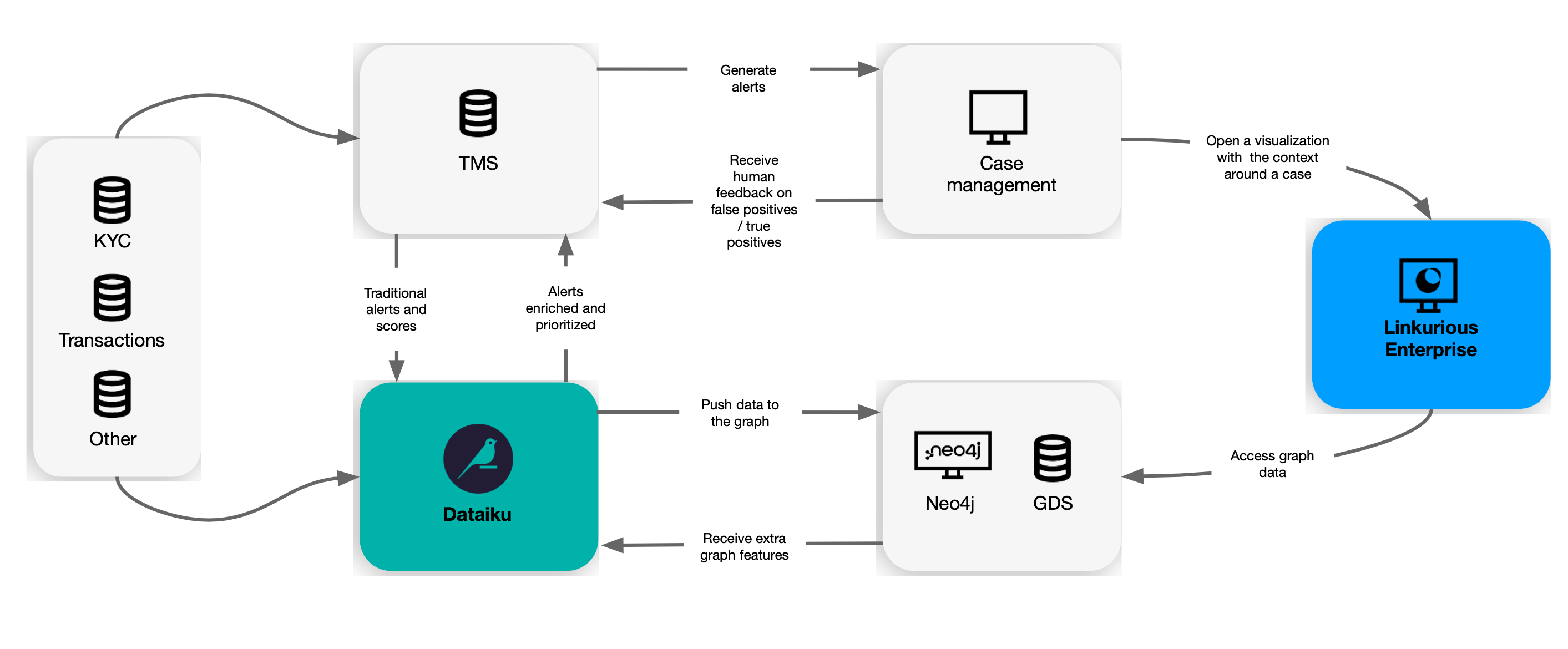
Combining business rules and machine learning algorithms in Dataiku
First, Dataiku can pull in data from your different datasets. You can have multiple different datasets in a single project. You can then do all sorts of things with your data within Dataiku: process it in smaller data increments, group it, etc. You can also write business rules using simple Python scripts.
In parallel to those business rules, you can use machine learning algorithms. In our recent webinar with Dataiku, we used an isolation forest algorithm for anomaly detection. You can also set up an algorithm to rank or tier the alerts of the business rules or the machine learning model. This helps determine based on historical trends which alerts are most likely to end up being escalated as a SAR - effectively helping reduce false positives.
It’s easy for teams to set up and design these machine learning algorithms within the Dataiku interface. And these pieces of enrichment can then be passed into the Neo4j database using connectors.
Data exploration and investigation in Linkurious Enterprise
The same Neo4j database used in Dataiku can be directly accessed in Linkurious Enterprise. Neo4j acts as the shared, connective element between the two softwares. Connecting the two environments is as easy as plugging Linkurious Enterprise into the Neo4j instance connected to Dataiku. Once Linkurious Enterprise is connected to that instance, the data that will flow through Dataiku is also accessible via the Linkurious Enterprise interface. Now, any transactions being added to Dataiku, or new risk scores being calculated by Dataiku’s machine learning, will show up in Linkurious Enterprise.
As an analyst doing triage, you can now see those open cases directly in Linkurious Enterprise. You can open an alert and the software will immediately give you context around that alert. You can see not just the transaction but also the other elements that are surrounding it. The ability to easily explore the data in a highly visual and easy to use interface enables both technical and non technical users to lead to super efficient investigations for even the most complex cases.
Go further with graph and AI for AML
To learn more about how graph technology and artificial intelligence combined can enhance your AML systems to improve efficiency, watch our recent webinar. We demonstrate exactly how Linkurious Enterprise and Dataiku work together to more effectively detect false negatives, reduce false positives, and accelerate AML investigations. You can watch the replay here.
(1) https://www.reuters.com/article/bc-finreg-laundering-detecting-idUSKCN1GP2NV
(2) https://www.pymnts.com/news/regulation/2023/report-2022-saw-50-spike-in-money-laundering-fines/
A spotlight on graph technology directly in your inbox.