Detecting synthetic identity fraud using graph technology
Synthetic identity fraud is the fastest growing financial crime in the US according to estimates by McKinsey (1), but its reach isn't limited to one country: it happens around the world. It's estimated that $20 to $40 billion is lost to synthetic identity fraud each year (2).
Synthetic identity fraud (also called synthetic fraud, synthetic ID fraud, or synthetic identity theft) negatively impacts banks, financial institutions and public organizations, as well as the individuals whose data may have been compromised by fraudsters. It’s also a notoriously difficult type of fraud to detect.
This article defines what synthetic identity fraud is and why criminals have been getting away with it. It also explores how graph visualization and analytics technology can be a high-performing asset in your arsenal to combat synthetic fraud.
A synthetic identity is a false identity mixing real and fake personal information. It may include a real social security number (SSN) and a fake name, for example. This information together does not belong to any one real person.
This differentiates synthetic fraud from identity theft. In the latter, a real individual’s actual personal credentials are stolen and used to open or access accounts in order to commit acts of fraud.
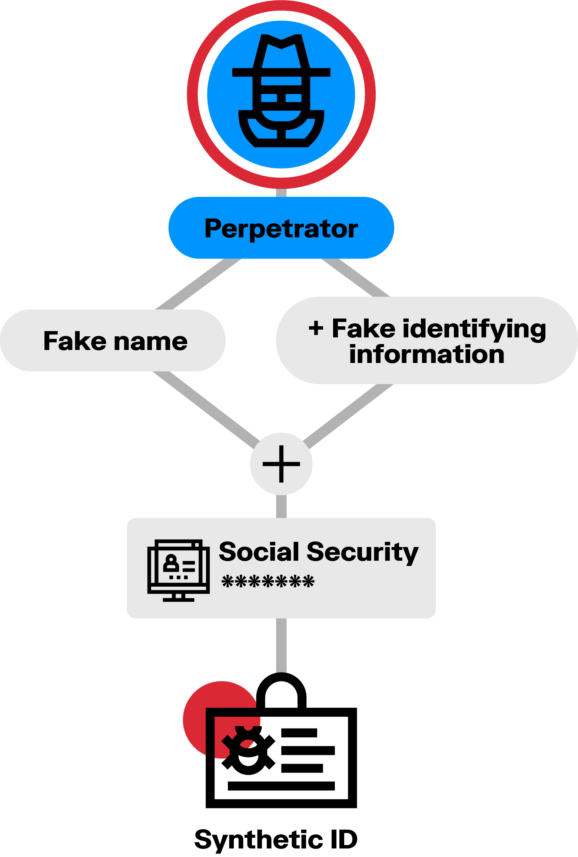
Synthetic identity fraud is an increasingly common problem for several reasons.
- First, security improvements in physical credit cards have sent fraudsters searching for new criminal avenues. Creating a synthetic identity is a relatively cheap and low-risk way to steal money online.
- Second, financial services are increasingly digitized. With more transactions taking place online, risks are evolving. There are more opportunities for bad actors to hide behind fake or synthetic identities.
- Finally, numerous data breaches in recent years have led to PII being readily available for purchase on the dark web.
Compounding this latter phenomenon are changes in the ways social security numbers are used and issued in the United States (where synthetic ID fraud is a bigger problem than elsewhere in the world). They are used by both the government and private businesses to assess the legitimacy of an individual. And in 2011, the social security administration changed how they issue SSNs, which now no longer contain geographical information, making it more difficult for organizations to detect discrepancies.
The first step in any synthetic fraud scheme is for the fraudster to create their new fake identity. Often they will do this using personal identifiable information (PII) they’ve gotten off the dark web. This information is easy to obtain. Synthetic identity fraud has grown so much that some criminals have set up fraud-as-a-service businesses, selling SSNs, credit card numbers, and other private data to help others commit fraud.
Commonly, fraudsters will use their synthetic identity to defraud financial services providers. In these cases, the next step is to apply for credit. Usually, this new person who has appeared out of nowhere, with no financial history, will be rejected. But by simply applying for credit, they’ve created a credit profile that they can now build on. The fraudster will continue applying for credit until they are finally approved by a lender.
Now, they can build on that to improve their credit score, which in turn enables them to apply for more and more. The fraudster will accelerate their positive credit history using their synthetic identity. They may also do what is called “piggybacking”: adding their synthetic ID as an authorized user to an account with a good credit score, which they then benefit from.
Using their good credit score, the fraudster can secure more and more credit. Eventually, they max out their credit and “bust out”, disappearing completely.
A fraudster may spend years building synthetic identities before applying for a credit line and disappearing.
It's worth noting that fraudsters can and do use synthetic identities in other types of criminal schemes as a convenient way to hide their identities and cover their trail. These might include:
- Romance scams
- Mule schemes
- Money laundering
- Illegal arms dealing
- Human trafficking
- Terrorism
Synthetic identity fraud has a serious impact on its victims. For people whose identities have been stolen to create synthetic identities, the financial impacts can be long lasting and difficult to manage. Children are often impacted by SSN theft, which they don’t discover until they are 18 and try to take out a student loan, for example. Vulnerable people such as the elderly or the homeless are also frequent victims. They are then left with the task of cleaning up their credit scores, left in shambles by the fraudster.
On the business side, synthetic identity fraud can be expensive. Aité Group found that US credit card accounts lost some $820 million to synthetic identity fraud in 2018 (3) - an amount that Aité projected would only increase. Managing the consequences of synthetic fraud also costs financial institutions time and resources.
Synthetic identities often appear and behave like legitimate accounts, making them difficult to detect using conventional methods. The best time to uncover a fraudster using a synthetic ID is during the verification process when they apply for credit. But it's estimated that 95% of synthetic identities are not detected during the onboarding process (2). Indeed, it's difficult to catch someone using a fake identity when these fraudsters appear so much like honest customers.
Graph analytics, or link analysis, is a powerful tool for the task. In a graph technology model, data is structured as a network. Individual data points are stored as nodes, which are connected to each other by edges, representing the relationships between them.
A graph analytics approach like Linkurious Enterprise is able to combine data from multiple sources - like customer databases and external sources, for example - so you have access to all your data in one place. You can then quickly search and explore that data as a network and leverage powerful analytics to automatically detect suspicious connections in your data to quickly uncover fraudulent patterns at scale.
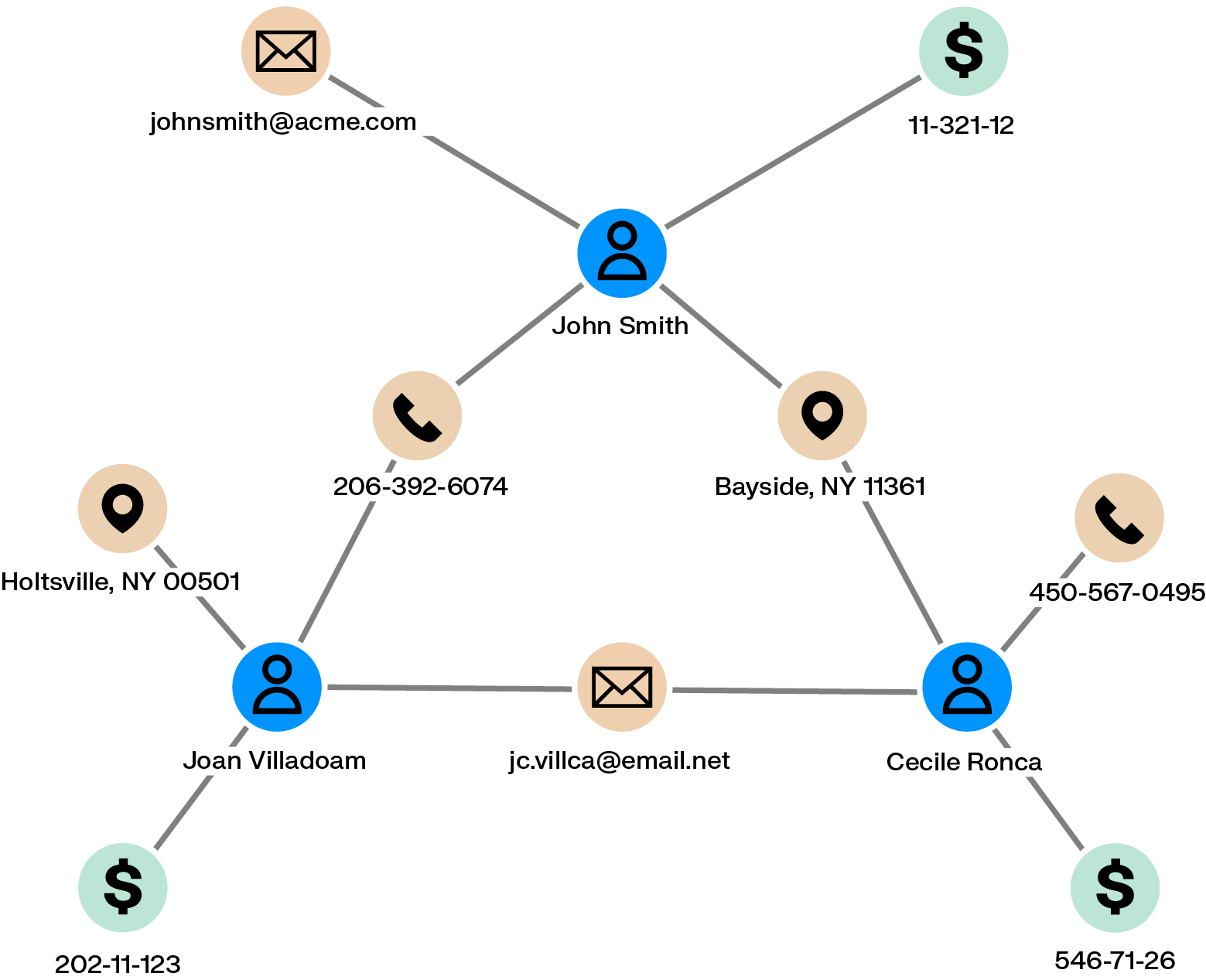
In the case of synthetic identity fraud, you can quickly see if there are pieces of data shared by multiple applicants or account holders. Several individuals interconnected across personally identifiable information such as an address, a phone number, a date of birth, an address, etc. may indicate a ring of fraudsters operating with synthetic identities. Catching them before they act mitigates damage and saves time for financial institutions.
You can automatically detect synthetic identities using the Alerts feature in Linkurious Enterprise:
Graph analytics and visualization is a powerful solution for detecting not only synthetic identity fraud, but many other types of complex fraud. See how graph analytics can speed up fraud investigations and improve fraud detection in our in-depth ebook.
(1) https://www.mckinsey.com/business-functions/risk-and-resilience/our-insights/fighting-back-against-synthetic-identity-fraud
(2) https://legal.thomsonreuters.com/en/insights/articles/trends-in-synthetic-identity-fraud
(3) https://legal.thomsonreuters.com/en/insights/articles/synthetic-identity-fraud
A spotlight on graph technology directly in your inbox.